Coefficient of determination, why?
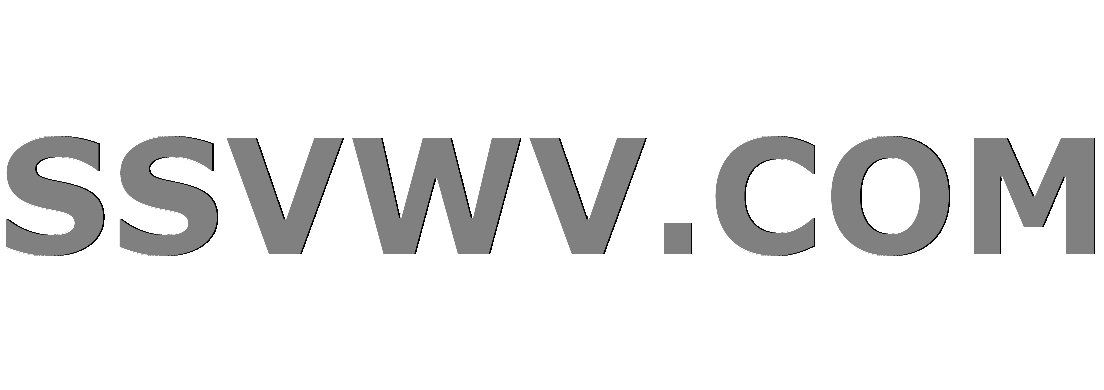
Multi tool use
$begingroup$
I mean it is written in a book "Statistics for Management and Economics", that coefficient of determination is coefficient of correlation squared. Well, am I the only one to whom this is surprising fact as he expected something more clear or natural?? I mean, if someone can present me the proof why, or why some other, more natural things do not work, like I don't know, absolute value of the coefficient of correlation or something similar to Chebyshev theorem ($1-text{coefficient of correlation}$)?
statistics
$endgroup$
add a comment |
$begingroup$
I mean it is written in a book "Statistics for Management and Economics", that coefficient of determination is coefficient of correlation squared. Well, am I the only one to whom this is surprising fact as he expected something more clear or natural?? I mean, if someone can present me the proof why, or why some other, more natural things do not work, like I don't know, absolute value of the coefficient of correlation or something similar to Chebyshev theorem ($1-text{coefficient of correlation}$)?
statistics
$endgroup$
1
$begingroup$
The title of the question is very confusing, could you please edit it so it is more clear?
$endgroup$
– Yuriy S
Jan 17 at 11:35
add a comment |
$begingroup$
I mean it is written in a book "Statistics for Management and Economics", that coefficient of determination is coefficient of correlation squared. Well, am I the only one to whom this is surprising fact as he expected something more clear or natural?? I mean, if someone can present me the proof why, or why some other, more natural things do not work, like I don't know, absolute value of the coefficient of correlation or something similar to Chebyshev theorem ($1-text{coefficient of correlation}$)?
statistics
$endgroup$
I mean it is written in a book "Statistics for Management and Economics", that coefficient of determination is coefficient of correlation squared. Well, am I the only one to whom this is surprising fact as he expected something more clear or natural?? I mean, if someone can present me the proof why, or why some other, more natural things do not work, like I don't know, absolute value of the coefficient of correlation or something similar to Chebyshev theorem ($1-text{coefficient of correlation}$)?
statistics
statistics
edited Jan 17 at 11:03
Christoph
12k1642
12k1642
asked Jan 17 at 10:54
nikolanikola
641314
641314
1
$begingroup$
The title of the question is very confusing, could you please edit it so it is more clear?
$endgroup$
– Yuriy S
Jan 17 at 11:35
add a comment |
1
$begingroup$
The title of the question is very confusing, could you please edit it so it is more clear?
$endgroup$
– Yuriy S
Jan 17 at 11:35
1
1
$begingroup$
The title of the question is very confusing, could you please edit it so it is more clear?
$endgroup$
– Yuriy S
Jan 17 at 11:35
$begingroup$
The title of the question is very confusing, could you please edit it so it is more clear?
$endgroup$
– Yuriy S
Jan 17 at 11:35
add a comment |
1 Answer
1
active
oldest
votes
$begingroup$
Suppose you have $n$ paired observations $(x_i,y_i)$ on $(x,y)$ and you want to predict $y$ on the basis of $x$.
You consider the prediction model $$y=phi(x)+e$$ where $phi$ is the part of $y$ explained by $x$ through $phi$ and $e$ is the unexplained part.
Suppose you see from the scatter plot of $x$ and $y$ that $phi$ is more or less linear.
So you choose $$phi(x)=a+bx$$
Then the least square linear predictor of $y$ obtained on the basis of $x$ is $$hat y=hat a+hat b x$$, where
$$hat a=bar y-hat bbar xquad,quad hat b=frac{operatorname{cov}(x,y)}{operatorname{var}(x)}$$
It can be shown that
begin{align}
operatorname{var}(hat y)&=operatorname{var}(hat a+hat b x)
\&=hat b^2operatorname{var}(x)
\&=r^2 operatorname{var}(y)
end{align}
, where $r$ is the correlation coefficient between $x$ and $y$.
A measure of efficacy of the predictor $phi$ is given by the proportion of variation in $y$ explained by $phi$, i.e., $$frac{operatorname{var}(hat y)}{operatorname{var}(y)}=r^2$$, which is termed as coefficient of determination.
Of course, the coefficient of determination is numerically equal to the square of the correlation coefficient, but that is hardly a definition or a motivation for the former.
When $r^2=0$ the linear prediction of $y$ obtained on the basis of $x$ is worst, and when $r^2=1$ the prediction is perfect as $phi$ explains the variability in $y$ completely.
For more details, the following threads might be helpful:
Correlation Coefficient and Determination Coefficient
https://stats.stackexchange.com/questions/123651/geometric-interpretation-of-multiple-correlation-coefficient-r-and-coefficient?noredirect=1&lq=1
https://stats.stackexchange.com/questions/1447/coefficient-of-determination-r2-i-have-never-fully-grasped-the-interpretat?noredirect=1&lq=1.
$endgroup$
$begingroup$
Excellent answer
$endgroup$
– lux
Jan 18 at 1:07
add a comment |
Your Answer
StackExchange.ifUsing("editor", function () {
return StackExchange.using("mathjaxEditing", function () {
StackExchange.MarkdownEditor.creationCallbacks.add(function (editor, postfix) {
StackExchange.mathjaxEditing.prepareWmdForMathJax(editor, postfix, [["$", "$"], ["\\(","\\)"]]);
});
});
}, "mathjax-editing");
StackExchange.ready(function() {
var channelOptions = {
tags: "".split(" "),
id: "69"
};
initTagRenderer("".split(" "), "".split(" "), channelOptions);
StackExchange.using("externalEditor", function() {
// Have to fire editor after snippets, if snippets enabled
if (StackExchange.settings.snippets.snippetsEnabled) {
StackExchange.using("snippets", function() {
createEditor();
});
}
else {
createEditor();
}
});
function createEditor() {
StackExchange.prepareEditor({
heartbeatType: 'answer',
autoActivateHeartbeat: false,
convertImagesToLinks: true,
noModals: true,
showLowRepImageUploadWarning: true,
reputationToPostImages: 10,
bindNavPrevention: true,
postfix: "",
imageUploader: {
brandingHtml: "Powered by u003ca class="icon-imgur-white" href="https://imgur.com/"u003eu003c/au003e",
contentPolicyHtml: "User contributions licensed under u003ca href="https://creativecommons.org/licenses/by-sa/3.0/"u003ecc by-sa 3.0 with attribution requiredu003c/au003e u003ca href="https://stackoverflow.com/legal/content-policy"u003e(content policy)u003c/au003e",
allowUrls: true
},
noCode: true, onDemand: true,
discardSelector: ".discard-answer"
,immediatelyShowMarkdownHelp:true
});
}
});
Sign up or log in
StackExchange.ready(function () {
StackExchange.helpers.onClickDraftSave('#login-link');
});
Sign up using Google
Sign up using Facebook
Sign up using Email and Password
Post as a guest
Required, but never shown
StackExchange.ready(
function () {
StackExchange.openid.initPostLogin('.new-post-login', 'https%3a%2f%2fmath.stackexchange.com%2fquestions%2f3076842%2fcoefficient-of-determination-why%23new-answer', 'question_page');
}
);
Post as a guest
Required, but never shown
1 Answer
1
active
oldest
votes
1 Answer
1
active
oldest
votes
active
oldest
votes
active
oldest
votes
$begingroup$
Suppose you have $n$ paired observations $(x_i,y_i)$ on $(x,y)$ and you want to predict $y$ on the basis of $x$.
You consider the prediction model $$y=phi(x)+e$$ where $phi$ is the part of $y$ explained by $x$ through $phi$ and $e$ is the unexplained part.
Suppose you see from the scatter plot of $x$ and $y$ that $phi$ is more or less linear.
So you choose $$phi(x)=a+bx$$
Then the least square linear predictor of $y$ obtained on the basis of $x$ is $$hat y=hat a+hat b x$$, where
$$hat a=bar y-hat bbar xquad,quad hat b=frac{operatorname{cov}(x,y)}{operatorname{var}(x)}$$
It can be shown that
begin{align}
operatorname{var}(hat y)&=operatorname{var}(hat a+hat b x)
\&=hat b^2operatorname{var}(x)
\&=r^2 operatorname{var}(y)
end{align}
, where $r$ is the correlation coefficient between $x$ and $y$.
A measure of efficacy of the predictor $phi$ is given by the proportion of variation in $y$ explained by $phi$, i.e., $$frac{operatorname{var}(hat y)}{operatorname{var}(y)}=r^2$$, which is termed as coefficient of determination.
Of course, the coefficient of determination is numerically equal to the square of the correlation coefficient, but that is hardly a definition or a motivation for the former.
When $r^2=0$ the linear prediction of $y$ obtained on the basis of $x$ is worst, and when $r^2=1$ the prediction is perfect as $phi$ explains the variability in $y$ completely.
For more details, the following threads might be helpful:
Correlation Coefficient and Determination Coefficient
https://stats.stackexchange.com/questions/123651/geometric-interpretation-of-multiple-correlation-coefficient-r-and-coefficient?noredirect=1&lq=1
https://stats.stackexchange.com/questions/1447/coefficient-of-determination-r2-i-have-never-fully-grasped-the-interpretat?noredirect=1&lq=1.
$endgroup$
$begingroup$
Excellent answer
$endgroup$
– lux
Jan 18 at 1:07
add a comment |
$begingroup$
Suppose you have $n$ paired observations $(x_i,y_i)$ on $(x,y)$ and you want to predict $y$ on the basis of $x$.
You consider the prediction model $$y=phi(x)+e$$ where $phi$ is the part of $y$ explained by $x$ through $phi$ and $e$ is the unexplained part.
Suppose you see from the scatter plot of $x$ and $y$ that $phi$ is more or less linear.
So you choose $$phi(x)=a+bx$$
Then the least square linear predictor of $y$ obtained on the basis of $x$ is $$hat y=hat a+hat b x$$, where
$$hat a=bar y-hat bbar xquad,quad hat b=frac{operatorname{cov}(x,y)}{operatorname{var}(x)}$$
It can be shown that
begin{align}
operatorname{var}(hat y)&=operatorname{var}(hat a+hat b x)
\&=hat b^2operatorname{var}(x)
\&=r^2 operatorname{var}(y)
end{align}
, where $r$ is the correlation coefficient between $x$ and $y$.
A measure of efficacy of the predictor $phi$ is given by the proportion of variation in $y$ explained by $phi$, i.e., $$frac{operatorname{var}(hat y)}{operatorname{var}(y)}=r^2$$, which is termed as coefficient of determination.
Of course, the coefficient of determination is numerically equal to the square of the correlation coefficient, but that is hardly a definition or a motivation for the former.
When $r^2=0$ the linear prediction of $y$ obtained on the basis of $x$ is worst, and when $r^2=1$ the prediction is perfect as $phi$ explains the variability in $y$ completely.
For more details, the following threads might be helpful:
Correlation Coefficient and Determination Coefficient
https://stats.stackexchange.com/questions/123651/geometric-interpretation-of-multiple-correlation-coefficient-r-and-coefficient?noredirect=1&lq=1
https://stats.stackexchange.com/questions/1447/coefficient-of-determination-r2-i-have-never-fully-grasped-the-interpretat?noredirect=1&lq=1.
$endgroup$
$begingroup$
Excellent answer
$endgroup$
– lux
Jan 18 at 1:07
add a comment |
$begingroup$
Suppose you have $n$ paired observations $(x_i,y_i)$ on $(x,y)$ and you want to predict $y$ on the basis of $x$.
You consider the prediction model $$y=phi(x)+e$$ where $phi$ is the part of $y$ explained by $x$ through $phi$ and $e$ is the unexplained part.
Suppose you see from the scatter plot of $x$ and $y$ that $phi$ is more or less linear.
So you choose $$phi(x)=a+bx$$
Then the least square linear predictor of $y$ obtained on the basis of $x$ is $$hat y=hat a+hat b x$$, where
$$hat a=bar y-hat bbar xquad,quad hat b=frac{operatorname{cov}(x,y)}{operatorname{var}(x)}$$
It can be shown that
begin{align}
operatorname{var}(hat y)&=operatorname{var}(hat a+hat b x)
\&=hat b^2operatorname{var}(x)
\&=r^2 operatorname{var}(y)
end{align}
, where $r$ is the correlation coefficient between $x$ and $y$.
A measure of efficacy of the predictor $phi$ is given by the proportion of variation in $y$ explained by $phi$, i.e., $$frac{operatorname{var}(hat y)}{operatorname{var}(y)}=r^2$$, which is termed as coefficient of determination.
Of course, the coefficient of determination is numerically equal to the square of the correlation coefficient, but that is hardly a definition or a motivation for the former.
When $r^2=0$ the linear prediction of $y$ obtained on the basis of $x$ is worst, and when $r^2=1$ the prediction is perfect as $phi$ explains the variability in $y$ completely.
For more details, the following threads might be helpful:
Correlation Coefficient and Determination Coefficient
https://stats.stackexchange.com/questions/123651/geometric-interpretation-of-multiple-correlation-coefficient-r-and-coefficient?noredirect=1&lq=1
https://stats.stackexchange.com/questions/1447/coefficient-of-determination-r2-i-have-never-fully-grasped-the-interpretat?noredirect=1&lq=1.
$endgroup$
Suppose you have $n$ paired observations $(x_i,y_i)$ on $(x,y)$ and you want to predict $y$ on the basis of $x$.
You consider the prediction model $$y=phi(x)+e$$ where $phi$ is the part of $y$ explained by $x$ through $phi$ and $e$ is the unexplained part.
Suppose you see from the scatter plot of $x$ and $y$ that $phi$ is more or less linear.
So you choose $$phi(x)=a+bx$$
Then the least square linear predictor of $y$ obtained on the basis of $x$ is $$hat y=hat a+hat b x$$, where
$$hat a=bar y-hat bbar xquad,quad hat b=frac{operatorname{cov}(x,y)}{operatorname{var}(x)}$$
It can be shown that
begin{align}
operatorname{var}(hat y)&=operatorname{var}(hat a+hat b x)
\&=hat b^2operatorname{var}(x)
\&=r^2 operatorname{var}(y)
end{align}
, where $r$ is the correlation coefficient between $x$ and $y$.
A measure of efficacy of the predictor $phi$ is given by the proportion of variation in $y$ explained by $phi$, i.e., $$frac{operatorname{var}(hat y)}{operatorname{var}(y)}=r^2$$, which is termed as coefficient of determination.
Of course, the coefficient of determination is numerically equal to the square of the correlation coefficient, but that is hardly a definition or a motivation for the former.
When $r^2=0$ the linear prediction of $y$ obtained on the basis of $x$ is worst, and when $r^2=1$ the prediction is perfect as $phi$ explains the variability in $y$ completely.
For more details, the following threads might be helpful:
Correlation Coefficient and Determination Coefficient
https://stats.stackexchange.com/questions/123651/geometric-interpretation-of-multiple-correlation-coefficient-r-and-coefficient?noredirect=1&lq=1
https://stats.stackexchange.com/questions/1447/coefficient-of-determination-r2-i-have-never-fully-grasped-the-interpretat?noredirect=1&lq=1.
answered Jan 17 at 13:02


StubbornAtomStubbornAtom
5,98311238
5,98311238
$begingroup$
Excellent answer
$endgroup$
– lux
Jan 18 at 1:07
add a comment |
$begingroup$
Excellent answer
$endgroup$
– lux
Jan 18 at 1:07
$begingroup$
Excellent answer
$endgroup$
– lux
Jan 18 at 1:07
$begingroup$
Excellent answer
$endgroup$
– lux
Jan 18 at 1:07
add a comment |
Thanks for contributing an answer to Mathematics Stack Exchange!
- Please be sure to answer the question. Provide details and share your research!
But avoid …
- Asking for help, clarification, or responding to other answers.
- Making statements based on opinion; back them up with references or personal experience.
Use MathJax to format equations. MathJax reference.
To learn more, see our tips on writing great answers.
Sign up or log in
StackExchange.ready(function () {
StackExchange.helpers.onClickDraftSave('#login-link');
});
Sign up using Google
Sign up using Facebook
Sign up using Email and Password
Post as a guest
Required, but never shown
StackExchange.ready(
function () {
StackExchange.openid.initPostLogin('.new-post-login', 'https%3a%2f%2fmath.stackexchange.com%2fquestions%2f3076842%2fcoefficient-of-determination-why%23new-answer', 'question_page');
}
);
Post as a guest
Required, but never shown
Sign up or log in
StackExchange.ready(function () {
StackExchange.helpers.onClickDraftSave('#login-link');
});
Sign up using Google
Sign up using Facebook
Sign up using Email and Password
Post as a guest
Required, but never shown
Sign up or log in
StackExchange.ready(function () {
StackExchange.helpers.onClickDraftSave('#login-link');
});
Sign up using Google
Sign up using Facebook
Sign up using Email and Password
Post as a guest
Required, but never shown
Sign up or log in
StackExchange.ready(function () {
StackExchange.helpers.onClickDraftSave('#login-link');
});
Sign up using Google
Sign up using Facebook
Sign up using Email and Password
Sign up using Google
Sign up using Facebook
Sign up using Email and Password
Post as a guest
Required, but never shown
Required, but never shown
Required, but never shown
Required, but never shown
Required, but never shown
Required, but never shown
Required, but never shown
Required, but never shown
Required, but never shown
Z2McOW6ND
1
$begingroup$
The title of the question is very confusing, could you please edit it so it is more clear?
$endgroup$
– Yuriy S
Jan 17 at 11:35