How to easily see the time integral of a Brownian motion is normally distributed?
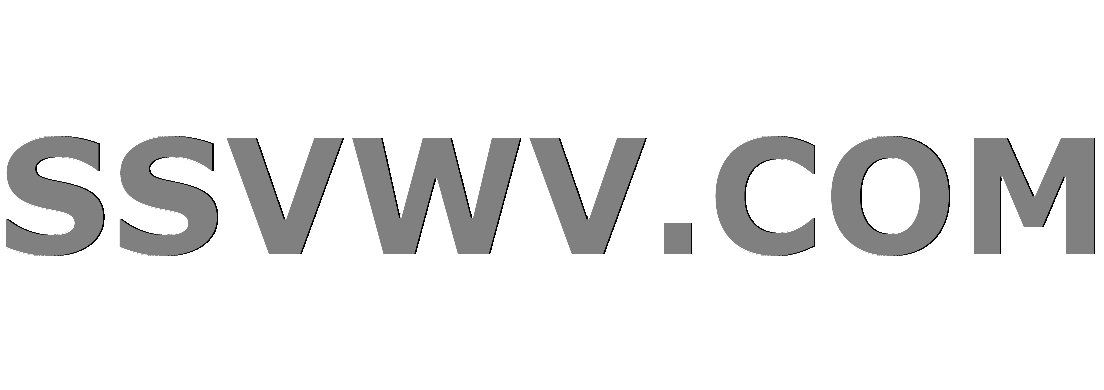
Multi tool use
$begingroup$
It's well known that $X_t:=int_0^tB_tau dtau$ where ${B_tau}$ is a 1D standard Brownian motion is distributed as $N(0, t^3/3)$. Is there any "immediate" way to see this fact?
The easiest one I can get: discretise $X_t$ into Riemannian sum, and break each $B_{tau_i}$ into independent increments over tiny intervals, then we see the sum is just a linear sum of independent normal distributions, then take the limit and use convergence in distribution to conclude.
I wouldn't say this is hard, but this is in no way trivial or immediate either. Can we somehow see this fact without any effort or whatsoever? Thanks.
probability probability-theory stochastic-processes brownian-motion
$endgroup$
|
show 1 more comment
$begingroup$
It's well known that $X_t:=int_0^tB_tau dtau$ where ${B_tau}$ is a 1D standard Brownian motion is distributed as $N(0, t^3/3)$. Is there any "immediate" way to see this fact?
The easiest one I can get: discretise $X_t$ into Riemannian sum, and break each $B_{tau_i}$ into independent increments over tiny intervals, then we see the sum is just a linear sum of independent normal distributions, then take the limit and use convergence in distribution to conclude.
I wouldn't say this is hard, but this is in no way trivial or immediate either. Can we somehow see this fact without any effort or whatsoever? Thanks.
probability probability-theory stochastic-processes brownian-motion
$endgroup$
1
$begingroup$
Writing the integral as limit of Riemann sums is ths natural easiest way to prove this.
$endgroup$
– Kavi Rama Murthy
Jan 16 at 8:26
$begingroup$
@KaviRamaMurthy Ok, but I'm still expecting something easier, like a "continuous" argument (cleverly determine its characterisitc function, so to speak). Discretisation looks non-tidy, albeit natural.
$endgroup$
– Vim
Jan 16 at 8:29
$begingroup$
Why do you have to breat $B_{tau_i}$ into tiny intervals...? The random vector $(B_{t_1},ldots,B_{t_n})$ is Gaussian for any choice of $t_i$ (as $B$ is a Gaussian process), and hence $$sum_{i=1}^n c_i B_{t_i}$$ is Gaussian for any choice of constants $c_i$.
$endgroup$
– saz
Jan 16 at 8:57
$begingroup$
@saz you're definitely correct. It's just that estimating the variance of independent sums is easier.
$endgroup$
– Vim
Jan 16 at 12:53
1
$begingroup$
@Vim Well, the expectation as well as the variance can be calculated using Fubini's theorem... that's not really a problem. From my point of view, the key point is to show that $X_t$ is Gaussian. By the way, I don't think that it possible to get rid of the discretisation - simply because the integral is defined as the limit of the discretisations. Obviously, you can apply some "big" result and hide the discretisation there... but that doesn't make the proof "easier", in my oppinion.
$endgroup$
– saz
Jan 16 at 14:03
|
show 1 more comment
$begingroup$
It's well known that $X_t:=int_0^tB_tau dtau$ where ${B_tau}$ is a 1D standard Brownian motion is distributed as $N(0, t^3/3)$. Is there any "immediate" way to see this fact?
The easiest one I can get: discretise $X_t$ into Riemannian sum, and break each $B_{tau_i}$ into independent increments over tiny intervals, then we see the sum is just a linear sum of independent normal distributions, then take the limit and use convergence in distribution to conclude.
I wouldn't say this is hard, but this is in no way trivial or immediate either. Can we somehow see this fact without any effort or whatsoever? Thanks.
probability probability-theory stochastic-processes brownian-motion
$endgroup$
It's well known that $X_t:=int_0^tB_tau dtau$ where ${B_tau}$ is a 1D standard Brownian motion is distributed as $N(0, t^3/3)$. Is there any "immediate" way to see this fact?
The easiest one I can get: discretise $X_t$ into Riemannian sum, and break each $B_{tau_i}$ into independent increments over tiny intervals, then we see the sum is just a linear sum of independent normal distributions, then take the limit and use convergence in distribution to conclude.
I wouldn't say this is hard, but this is in no way trivial or immediate either. Can we somehow see this fact without any effort or whatsoever? Thanks.
probability probability-theory stochastic-processes brownian-motion
probability probability-theory stochastic-processes brownian-motion
asked Jan 16 at 8:24


VimVim
8,10031348
8,10031348
1
$begingroup$
Writing the integral as limit of Riemann sums is ths natural easiest way to prove this.
$endgroup$
– Kavi Rama Murthy
Jan 16 at 8:26
$begingroup$
@KaviRamaMurthy Ok, but I'm still expecting something easier, like a "continuous" argument (cleverly determine its characterisitc function, so to speak). Discretisation looks non-tidy, albeit natural.
$endgroup$
– Vim
Jan 16 at 8:29
$begingroup$
Why do you have to breat $B_{tau_i}$ into tiny intervals...? The random vector $(B_{t_1},ldots,B_{t_n})$ is Gaussian for any choice of $t_i$ (as $B$ is a Gaussian process), and hence $$sum_{i=1}^n c_i B_{t_i}$$ is Gaussian for any choice of constants $c_i$.
$endgroup$
– saz
Jan 16 at 8:57
$begingroup$
@saz you're definitely correct. It's just that estimating the variance of independent sums is easier.
$endgroup$
– Vim
Jan 16 at 12:53
1
$begingroup$
@Vim Well, the expectation as well as the variance can be calculated using Fubini's theorem... that's not really a problem. From my point of view, the key point is to show that $X_t$ is Gaussian. By the way, I don't think that it possible to get rid of the discretisation - simply because the integral is defined as the limit of the discretisations. Obviously, you can apply some "big" result and hide the discretisation there... but that doesn't make the proof "easier", in my oppinion.
$endgroup$
– saz
Jan 16 at 14:03
|
show 1 more comment
1
$begingroup$
Writing the integral as limit of Riemann sums is ths natural easiest way to prove this.
$endgroup$
– Kavi Rama Murthy
Jan 16 at 8:26
$begingroup$
@KaviRamaMurthy Ok, but I'm still expecting something easier, like a "continuous" argument (cleverly determine its characterisitc function, so to speak). Discretisation looks non-tidy, albeit natural.
$endgroup$
– Vim
Jan 16 at 8:29
$begingroup$
Why do you have to breat $B_{tau_i}$ into tiny intervals...? The random vector $(B_{t_1},ldots,B_{t_n})$ is Gaussian for any choice of $t_i$ (as $B$ is a Gaussian process), and hence $$sum_{i=1}^n c_i B_{t_i}$$ is Gaussian for any choice of constants $c_i$.
$endgroup$
– saz
Jan 16 at 8:57
$begingroup$
@saz you're definitely correct. It's just that estimating the variance of independent sums is easier.
$endgroup$
– Vim
Jan 16 at 12:53
1
$begingroup$
@Vim Well, the expectation as well as the variance can be calculated using Fubini's theorem... that's not really a problem. From my point of view, the key point is to show that $X_t$ is Gaussian. By the way, I don't think that it possible to get rid of the discretisation - simply because the integral is defined as the limit of the discretisations. Obviously, you can apply some "big" result and hide the discretisation there... but that doesn't make the proof "easier", in my oppinion.
$endgroup$
– saz
Jan 16 at 14:03
1
1
$begingroup$
Writing the integral as limit of Riemann sums is ths natural easiest way to prove this.
$endgroup$
– Kavi Rama Murthy
Jan 16 at 8:26
$begingroup$
Writing the integral as limit of Riemann sums is ths natural easiest way to prove this.
$endgroup$
– Kavi Rama Murthy
Jan 16 at 8:26
$begingroup$
@KaviRamaMurthy Ok, but I'm still expecting something easier, like a "continuous" argument (cleverly determine its characterisitc function, so to speak). Discretisation looks non-tidy, albeit natural.
$endgroup$
– Vim
Jan 16 at 8:29
$begingroup$
@KaviRamaMurthy Ok, but I'm still expecting something easier, like a "continuous" argument (cleverly determine its characterisitc function, so to speak). Discretisation looks non-tidy, albeit natural.
$endgroup$
– Vim
Jan 16 at 8:29
$begingroup$
Why do you have to breat $B_{tau_i}$ into tiny intervals...? The random vector $(B_{t_1},ldots,B_{t_n})$ is Gaussian for any choice of $t_i$ (as $B$ is a Gaussian process), and hence $$sum_{i=1}^n c_i B_{t_i}$$ is Gaussian for any choice of constants $c_i$.
$endgroup$
– saz
Jan 16 at 8:57
$begingroup$
Why do you have to breat $B_{tau_i}$ into tiny intervals...? The random vector $(B_{t_1},ldots,B_{t_n})$ is Gaussian for any choice of $t_i$ (as $B$ is a Gaussian process), and hence $$sum_{i=1}^n c_i B_{t_i}$$ is Gaussian for any choice of constants $c_i$.
$endgroup$
– saz
Jan 16 at 8:57
$begingroup$
@saz you're definitely correct. It's just that estimating the variance of independent sums is easier.
$endgroup$
– Vim
Jan 16 at 12:53
$begingroup$
@saz you're definitely correct. It's just that estimating the variance of independent sums is easier.
$endgroup$
– Vim
Jan 16 at 12:53
1
1
$begingroup$
@Vim Well, the expectation as well as the variance can be calculated using Fubini's theorem... that's not really a problem. From my point of view, the key point is to show that $X_t$ is Gaussian. By the way, I don't think that it possible to get rid of the discretisation - simply because the integral is defined as the limit of the discretisations. Obviously, you can apply some "big" result and hide the discretisation there... but that doesn't make the proof "easier", in my oppinion.
$endgroup$
– saz
Jan 16 at 14:03
$begingroup$
@Vim Well, the expectation as well as the variance can be calculated using Fubini's theorem... that's not really a problem. From my point of view, the key point is to show that $X_t$ is Gaussian. By the way, I don't think that it possible to get rid of the discretisation - simply because the integral is defined as the limit of the discretisations. Obviously, you can apply some "big" result and hide the discretisation there... but that doesn't make the proof "easier", in my oppinion.
$endgroup$
– saz
Jan 16 at 14:03
|
show 1 more comment
1 Answer
1
active
oldest
votes
$begingroup$
There are three things which need to be done:
- Check that $X_t$ is Gaussian.
- Compute its expectation.
- Compute its variance.
1. $X_t$ is Gaussian: It seems to me that the most natural (and also direct) way to prove this is an approximation by Riemann sums. Noting that
$$X_t^{(n)} := sum_{j=1}^n frac{1}{n} B_{t j/n} tag{1}$$
is Gaussian for each $t>0$ (because $(B_t)_{t geq 0}$ is a Gaussian process), we find that $$X_t = lim_{n to infty} X_t^{(n)}$$ is Gaussian as pointwise limit of Gaussian random variables. For an alternative reasoning see the very end of my answer.
2. Compute $mathbb{E}(X_t)$: Since $mathbb{E}(B_s)=0$ for all $s geq 0$, it follows that each $X_t^{(n)}$ (defined in $(1)$) has expectation zero, and hence its limit $X_t = lim_n X_t^{(n)}$ has expectation zero. Alternatively, we can apply Fubini's theorem:
$$mathbb{E}(X_t) = mathbb{E} left( int_0^t B_s , ds right) = int_0^t underbrace{mathbb{E}(B_s)}_{=0} , ds =0.$$
3. Compute $text{var}(X_t)$: Since we already know that $mathbb{E}(X_t)=0$, we clearly have $text{var}(X_t) = mathbb{E}(X_t^2)$. As
$$X_t^2 = int_0^t int_0^t B_s B_r , ds , dr$$
it follows from Fubini's theorem that
$$mathbb{E}(X_t^2) = int_0^t int_0^t mathbb{E}(B_s B_r) , ds , dr. tag{2}$$
By symmetry, we thus get
$$mathbb{E}(X_t^2) = 2 int_0^t int_0^r underbrace{mathbb{E}(B_s B_r)}_{min{s,r}=s} , ds , dr = 2 int_0^t int_0^r s , ds = frac{t^3}{3}$$
If you don't like symmetrization, then note that (2) implies
$$mathbb{E}(X_t^2) = int_0^t int_0^s underbrace{mathbb{E}(B_s B_r)}_{min{s,r}=s} , ds , dr + int_0^t int_s^t underbrace{mathbb{E}(B_s B_r)}_{min{s,r}=r} , ds , dr$$
and each of the integrals can be calculated explicitly using standard calculus.
Let me close this answer with a result which combines all the three steps into one.
Proposition: Let $(L_t)_{t geq 0}$ be a Lévy process with characteristic exponent $psi$, i.e. $$mathbb{E}exp(i xi L_t) = exp(-t psi(xi)), qquad t geq 0, xi in mathbb{R}. tag{3}$$ Then the characteristic function of $$X_t := int_0^t L_s , ds$$ equals $$phi(xi) = exp left(- int_0^t psi(xi s) , ds right), qquad xi in mathbb{R}.$$
If you are not familar with Lévy processes (that it, stochastic processes with independent and stationary increments), then you can just think of a Brownian motion; in this case $psi$ is given by $psi(xi) = xi^2/2$. Applying the proposition, we thus find that the characteristic function of $X_t = int_0^t B_s , ds$ equals $$exp left(- frac{t^3}{3} frac{xi^2}{2} right)$$ which is the characteristic function of $N(0,t^3/3)$, and so $X_t sim N(0,t^3/3)$.
Proof of the proposition: For fixed $n in mathbb{N}$ and $t>0$ set $t_j := t j/n$ for $j=1,ldots,n$, and set
$$phi_n(xi) := mathbb{E} exp left( i xi frac{1}{n} sum_{j=1}^n L_{t_j} right).$$
Denote by $mathcal{F}_t := sigma(L_s; s leq t)$ the canonical filtration of $(L_t)_{t geq 0}$. Using the tower property of the conditional expectation, we find
$$begin{align*} phi_n(xi) &= mathbb{E} bigg{ mathbb{E} bigg[ exp left( i xi frac{1}{n} sum_{j=1}^n L_{t_j} right) mid mathcal{F}_{t_{n-1}} bigg] bigg} \ &= mathbb{E} bigg{ exp left( i xi frac{1}{n} sum_{j=1}^{n-1} L_{t_j} right) mathbb{E} bigg[ exp left( i xi frac{1}{n} L_{t_n} right) mid mathcal{F}_{t_{n-1}} bigg] bigg} tag{4} end{align*}$$
Since $(L_t)_{t geq 0}$ has independent increments, we have
$$begin{align*} mathbb{E} bigg[ exp left( i xi frac{1}{n} L_{t_n} right) mid mathcal{F}_{t_{n-1}} bigg] &=exp(i xi/n L_{t_{n-1}}) mathbb{E} bigg[ exp left( i xi frac{1}{n} (L_{t_n}-L_{t_{n-1}}) right) mid mathcal{F}_{t_{n-1}} bigg] \ &= exp(i xi/n L_{t_{n-1}}) mathbb{E}expleft( i xi frac{1}{n} (L_{t_n}-L_{t_{n-1}}) right). end{align*}$$
Using that $(L_t)_{t geq 0}$ has stationary increments, i.e. $L_{t_n}-L_{t_{n-1}} sim L_{t_n-t_{n-1}}=L_{1/n}$, and using $(3)$ we thus get
$$ mathbb{E} bigg[ exp left( i xi frac{1}{n} L_{t_n} right) mid mathcal{F}_{t_{n-1}} bigg] = exp(i xi/n L_{t_{n-1}}) exp left(- frac{1}{n} psi left( frac{xi}{n} right) right).$$
Plugging this into $(4)$, we obtain that
$$phi_n(xi) = mathbb{E} bigg{ exp left( i xi frac{1}{n} sum_{j=1}^{n-2} L_{t_j} + i xi frac{2}{n} L_{t_{n-1}} right) exp left(- frac{1}{n} psi left( frac{xi}{n} right) right) .$$
Iterating this reasoning (i.e. next conditioning on $mathcal{F}_{t_{n-2}}$, then on $mathcal{F}_{t_{n-3}}$, ...) we conclude that
$$phi_n(xi) = exp left( - frac{1}{n} sum_{j=1}^n psi left( frac{j}{n} xi right) right).tag{5}$$
Finally, we note that
$$X_t stackrel{text{def}}{=} int_0^t L_s , ds = lim_{n to infty} frac{1}{n} sum_{j=1}^n L_{tj/n},$$
and thus, by letting $n to infty$ in (5), we get
$$mathbb{E}exp(i xi X_t) = exp left(- int_0^t psi(s xi) , ds right).$$
$endgroup$
$begingroup$
Thanks for such a detailed answer!
$endgroup$
– Vim
Jan 17 at 0:39
$begingroup$
@Vim You're welcome.
$endgroup$
– saz
Jan 17 at 7:30
add a comment |
Your Answer
StackExchange.ifUsing("editor", function () {
return StackExchange.using("mathjaxEditing", function () {
StackExchange.MarkdownEditor.creationCallbacks.add(function (editor, postfix) {
StackExchange.mathjaxEditing.prepareWmdForMathJax(editor, postfix, [["$", "$"], ["\\(","\\)"]]);
});
});
}, "mathjax-editing");
StackExchange.ready(function() {
var channelOptions = {
tags: "".split(" "),
id: "69"
};
initTagRenderer("".split(" "), "".split(" "), channelOptions);
StackExchange.using("externalEditor", function() {
// Have to fire editor after snippets, if snippets enabled
if (StackExchange.settings.snippets.snippetsEnabled) {
StackExchange.using("snippets", function() {
createEditor();
});
}
else {
createEditor();
}
});
function createEditor() {
StackExchange.prepareEditor({
heartbeatType: 'answer',
autoActivateHeartbeat: false,
convertImagesToLinks: true,
noModals: true,
showLowRepImageUploadWarning: true,
reputationToPostImages: 10,
bindNavPrevention: true,
postfix: "",
imageUploader: {
brandingHtml: "Powered by u003ca class="icon-imgur-white" href="https://imgur.com/"u003eu003c/au003e",
contentPolicyHtml: "User contributions licensed under u003ca href="https://creativecommons.org/licenses/by-sa/3.0/"u003ecc by-sa 3.0 with attribution requiredu003c/au003e u003ca href="https://stackoverflow.com/legal/content-policy"u003e(content policy)u003c/au003e",
allowUrls: true
},
noCode: true, onDemand: true,
discardSelector: ".discard-answer"
,immediatelyShowMarkdownHelp:true
});
}
});
Sign up or log in
StackExchange.ready(function () {
StackExchange.helpers.onClickDraftSave('#login-link');
});
Sign up using Google
Sign up using Facebook
Sign up using Email and Password
Post as a guest
Required, but never shown
StackExchange.ready(
function () {
StackExchange.openid.initPostLogin('.new-post-login', 'https%3a%2f%2fmath.stackexchange.com%2fquestions%2f3075464%2fhow-to-easily-see-the-time-integral-of-a-brownian-motion-is-normally-distributed%23new-answer', 'question_page');
}
);
Post as a guest
Required, but never shown
1 Answer
1
active
oldest
votes
1 Answer
1
active
oldest
votes
active
oldest
votes
active
oldest
votes
$begingroup$
There are three things which need to be done:
- Check that $X_t$ is Gaussian.
- Compute its expectation.
- Compute its variance.
1. $X_t$ is Gaussian: It seems to me that the most natural (and also direct) way to prove this is an approximation by Riemann sums. Noting that
$$X_t^{(n)} := sum_{j=1}^n frac{1}{n} B_{t j/n} tag{1}$$
is Gaussian for each $t>0$ (because $(B_t)_{t geq 0}$ is a Gaussian process), we find that $$X_t = lim_{n to infty} X_t^{(n)}$$ is Gaussian as pointwise limit of Gaussian random variables. For an alternative reasoning see the very end of my answer.
2. Compute $mathbb{E}(X_t)$: Since $mathbb{E}(B_s)=0$ for all $s geq 0$, it follows that each $X_t^{(n)}$ (defined in $(1)$) has expectation zero, and hence its limit $X_t = lim_n X_t^{(n)}$ has expectation zero. Alternatively, we can apply Fubini's theorem:
$$mathbb{E}(X_t) = mathbb{E} left( int_0^t B_s , ds right) = int_0^t underbrace{mathbb{E}(B_s)}_{=0} , ds =0.$$
3. Compute $text{var}(X_t)$: Since we already know that $mathbb{E}(X_t)=0$, we clearly have $text{var}(X_t) = mathbb{E}(X_t^2)$. As
$$X_t^2 = int_0^t int_0^t B_s B_r , ds , dr$$
it follows from Fubini's theorem that
$$mathbb{E}(X_t^2) = int_0^t int_0^t mathbb{E}(B_s B_r) , ds , dr. tag{2}$$
By symmetry, we thus get
$$mathbb{E}(X_t^2) = 2 int_0^t int_0^r underbrace{mathbb{E}(B_s B_r)}_{min{s,r}=s} , ds , dr = 2 int_0^t int_0^r s , ds = frac{t^3}{3}$$
If you don't like symmetrization, then note that (2) implies
$$mathbb{E}(X_t^2) = int_0^t int_0^s underbrace{mathbb{E}(B_s B_r)}_{min{s,r}=s} , ds , dr + int_0^t int_s^t underbrace{mathbb{E}(B_s B_r)}_{min{s,r}=r} , ds , dr$$
and each of the integrals can be calculated explicitly using standard calculus.
Let me close this answer with a result which combines all the three steps into one.
Proposition: Let $(L_t)_{t geq 0}$ be a Lévy process with characteristic exponent $psi$, i.e. $$mathbb{E}exp(i xi L_t) = exp(-t psi(xi)), qquad t geq 0, xi in mathbb{R}. tag{3}$$ Then the characteristic function of $$X_t := int_0^t L_s , ds$$ equals $$phi(xi) = exp left(- int_0^t psi(xi s) , ds right), qquad xi in mathbb{R}.$$
If you are not familar with Lévy processes (that it, stochastic processes with independent and stationary increments), then you can just think of a Brownian motion; in this case $psi$ is given by $psi(xi) = xi^2/2$. Applying the proposition, we thus find that the characteristic function of $X_t = int_0^t B_s , ds$ equals $$exp left(- frac{t^3}{3} frac{xi^2}{2} right)$$ which is the characteristic function of $N(0,t^3/3)$, and so $X_t sim N(0,t^3/3)$.
Proof of the proposition: For fixed $n in mathbb{N}$ and $t>0$ set $t_j := t j/n$ for $j=1,ldots,n$, and set
$$phi_n(xi) := mathbb{E} exp left( i xi frac{1}{n} sum_{j=1}^n L_{t_j} right).$$
Denote by $mathcal{F}_t := sigma(L_s; s leq t)$ the canonical filtration of $(L_t)_{t geq 0}$. Using the tower property of the conditional expectation, we find
$$begin{align*} phi_n(xi) &= mathbb{E} bigg{ mathbb{E} bigg[ exp left( i xi frac{1}{n} sum_{j=1}^n L_{t_j} right) mid mathcal{F}_{t_{n-1}} bigg] bigg} \ &= mathbb{E} bigg{ exp left( i xi frac{1}{n} sum_{j=1}^{n-1} L_{t_j} right) mathbb{E} bigg[ exp left( i xi frac{1}{n} L_{t_n} right) mid mathcal{F}_{t_{n-1}} bigg] bigg} tag{4} end{align*}$$
Since $(L_t)_{t geq 0}$ has independent increments, we have
$$begin{align*} mathbb{E} bigg[ exp left( i xi frac{1}{n} L_{t_n} right) mid mathcal{F}_{t_{n-1}} bigg] &=exp(i xi/n L_{t_{n-1}}) mathbb{E} bigg[ exp left( i xi frac{1}{n} (L_{t_n}-L_{t_{n-1}}) right) mid mathcal{F}_{t_{n-1}} bigg] \ &= exp(i xi/n L_{t_{n-1}}) mathbb{E}expleft( i xi frac{1}{n} (L_{t_n}-L_{t_{n-1}}) right). end{align*}$$
Using that $(L_t)_{t geq 0}$ has stationary increments, i.e. $L_{t_n}-L_{t_{n-1}} sim L_{t_n-t_{n-1}}=L_{1/n}$, and using $(3)$ we thus get
$$ mathbb{E} bigg[ exp left( i xi frac{1}{n} L_{t_n} right) mid mathcal{F}_{t_{n-1}} bigg] = exp(i xi/n L_{t_{n-1}}) exp left(- frac{1}{n} psi left( frac{xi}{n} right) right).$$
Plugging this into $(4)$, we obtain that
$$phi_n(xi) = mathbb{E} bigg{ exp left( i xi frac{1}{n} sum_{j=1}^{n-2} L_{t_j} + i xi frac{2}{n} L_{t_{n-1}} right) exp left(- frac{1}{n} psi left( frac{xi}{n} right) right) .$$
Iterating this reasoning (i.e. next conditioning on $mathcal{F}_{t_{n-2}}$, then on $mathcal{F}_{t_{n-3}}$, ...) we conclude that
$$phi_n(xi) = exp left( - frac{1}{n} sum_{j=1}^n psi left( frac{j}{n} xi right) right).tag{5}$$
Finally, we note that
$$X_t stackrel{text{def}}{=} int_0^t L_s , ds = lim_{n to infty} frac{1}{n} sum_{j=1}^n L_{tj/n},$$
and thus, by letting $n to infty$ in (5), we get
$$mathbb{E}exp(i xi X_t) = exp left(- int_0^t psi(s xi) , ds right).$$
$endgroup$
$begingroup$
Thanks for such a detailed answer!
$endgroup$
– Vim
Jan 17 at 0:39
$begingroup$
@Vim You're welcome.
$endgroup$
– saz
Jan 17 at 7:30
add a comment |
$begingroup$
There are three things which need to be done:
- Check that $X_t$ is Gaussian.
- Compute its expectation.
- Compute its variance.
1. $X_t$ is Gaussian: It seems to me that the most natural (and also direct) way to prove this is an approximation by Riemann sums. Noting that
$$X_t^{(n)} := sum_{j=1}^n frac{1}{n} B_{t j/n} tag{1}$$
is Gaussian for each $t>0$ (because $(B_t)_{t geq 0}$ is a Gaussian process), we find that $$X_t = lim_{n to infty} X_t^{(n)}$$ is Gaussian as pointwise limit of Gaussian random variables. For an alternative reasoning see the very end of my answer.
2. Compute $mathbb{E}(X_t)$: Since $mathbb{E}(B_s)=0$ for all $s geq 0$, it follows that each $X_t^{(n)}$ (defined in $(1)$) has expectation zero, and hence its limit $X_t = lim_n X_t^{(n)}$ has expectation zero. Alternatively, we can apply Fubini's theorem:
$$mathbb{E}(X_t) = mathbb{E} left( int_0^t B_s , ds right) = int_0^t underbrace{mathbb{E}(B_s)}_{=0} , ds =0.$$
3. Compute $text{var}(X_t)$: Since we already know that $mathbb{E}(X_t)=0$, we clearly have $text{var}(X_t) = mathbb{E}(X_t^2)$. As
$$X_t^2 = int_0^t int_0^t B_s B_r , ds , dr$$
it follows from Fubini's theorem that
$$mathbb{E}(X_t^2) = int_0^t int_0^t mathbb{E}(B_s B_r) , ds , dr. tag{2}$$
By symmetry, we thus get
$$mathbb{E}(X_t^2) = 2 int_0^t int_0^r underbrace{mathbb{E}(B_s B_r)}_{min{s,r}=s} , ds , dr = 2 int_0^t int_0^r s , ds = frac{t^3}{3}$$
If you don't like symmetrization, then note that (2) implies
$$mathbb{E}(X_t^2) = int_0^t int_0^s underbrace{mathbb{E}(B_s B_r)}_{min{s,r}=s} , ds , dr + int_0^t int_s^t underbrace{mathbb{E}(B_s B_r)}_{min{s,r}=r} , ds , dr$$
and each of the integrals can be calculated explicitly using standard calculus.
Let me close this answer with a result which combines all the three steps into one.
Proposition: Let $(L_t)_{t geq 0}$ be a Lévy process with characteristic exponent $psi$, i.e. $$mathbb{E}exp(i xi L_t) = exp(-t psi(xi)), qquad t geq 0, xi in mathbb{R}. tag{3}$$ Then the characteristic function of $$X_t := int_0^t L_s , ds$$ equals $$phi(xi) = exp left(- int_0^t psi(xi s) , ds right), qquad xi in mathbb{R}.$$
If you are not familar with Lévy processes (that it, stochastic processes with independent and stationary increments), then you can just think of a Brownian motion; in this case $psi$ is given by $psi(xi) = xi^2/2$. Applying the proposition, we thus find that the characteristic function of $X_t = int_0^t B_s , ds$ equals $$exp left(- frac{t^3}{3} frac{xi^2}{2} right)$$ which is the characteristic function of $N(0,t^3/3)$, and so $X_t sim N(0,t^3/3)$.
Proof of the proposition: For fixed $n in mathbb{N}$ and $t>0$ set $t_j := t j/n$ for $j=1,ldots,n$, and set
$$phi_n(xi) := mathbb{E} exp left( i xi frac{1}{n} sum_{j=1}^n L_{t_j} right).$$
Denote by $mathcal{F}_t := sigma(L_s; s leq t)$ the canonical filtration of $(L_t)_{t geq 0}$. Using the tower property of the conditional expectation, we find
$$begin{align*} phi_n(xi) &= mathbb{E} bigg{ mathbb{E} bigg[ exp left( i xi frac{1}{n} sum_{j=1}^n L_{t_j} right) mid mathcal{F}_{t_{n-1}} bigg] bigg} \ &= mathbb{E} bigg{ exp left( i xi frac{1}{n} sum_{j=1}^{n-1} L_{t_j} right) mathbb{E} bigg[ exp left( i xi frac{1}{n} L_{t_n} right) mid mathcal{F}_{t_{n-1}} bigg] bigg} tag{4} end{align*}$$
Since $(L_t)_{t geq 0}$ has independent increments, we have
$$begin{align*} mathbb{E} bigg[ exp left( i xi frac{1}{n} L_{t_n} right) mid mathcal{F}_{t_{n-1}} bigg] &=exp(i xi/n L_{t_{n-1}}) mathbb{E} bigg[ exp left( i xi frac{1}{n} (L_{t_n}-L_{t_{n-1}}) right) mid mathcal{F}_{t_{n-1}} bigg] \ &= exp(i xi/n L_{t_{n-1}}) mathbb{E}expleft( i xi frac{1}{n} (L_{t_n}-L_{t_{n-1}}) right). end{align*}$$
Using that $(L_t)_{t geq 0}$ has stationary increments, i.e. $L_{t_n}-L_{t_{n-1}} sim L_{t_n-t_{n-1}}=L_{1/n}$, and using $(3)$ we thus get
$$ mathbb{E} bigg[ exp left( i xi frac{1}{n} L_{t_n} right) mid mathcal{F}_{t_{n-1}} bigg] = exp(i xi/n L_{t_{n-1}}) exp left(- frac{1}{n} psi left( frac{xi}{n} right) right).$$
Plugging this into $(4)$, we obtain that
$$phi_n(xi) = mathbb{E} bigg{ exp left( i xi frac{1}{n} sum_{j=1}^{n-2} L_{t_j} + i xi frac{2}{n} L_{t_{n-1}} right) exp left(- frac{1}{n} psi left( frac{xi}{n} right) right) .$$
Iterating this reasoning (i.e. next conditioning on $mathcal{F}_{t_{n-2}}$, then on $mathcal{F}_{t_{n-3}}$, ...) we conclude that
$$phi_n(xi) = exp left( - frac{1}{n} sum_{j=1}^n psi left( frac{j}{n} xi right) right).tag{5}$$
Finally, we note that
$$X_t stackrel{text{def}}{=} int_0^t L_s , ds = lim_{n to infty} frac{1}{n} sum_{j=1}^n L_{tj/n},$$
and thus, by letting $n to infty$ in (5), we get
$$mathbb{E}exp(i xi X_t) = exp left(- int_0^t psi(s xi) , ds right).$$
$endgroup$
$begingroup$
Thanks for such a detailed answer!
$endgroup$
– Vim
Jan 17 at 0:39
$begingroup$
@Vim You're welcome.
$endgroup$
– saz
Jan 17 at 7:30
add a comment |
$begingroup$
There are three things which need to be done:
- Check that $X_t$ is Gaussian.
- Compute its expectation.
- Compute its variance.
1. $X_t$ is Gaussian: It seems to me that the most natural (and also direct) way to prove this is an approximation by Riemann sums. Noting that
$$X_t^{(n)} := sum_{j=1}^n frac{1}{n} B_{t j/n} tag{1}$$
is Gaussian for each $t>0$ (because $(B_t)_{t geq 0}$ is a Gaussian process), we find that $$X_t = lim_{n to infty} X_t^{(n)}$$ is Gaussian as pointwise limit of Gaussian random variables. For an alternative reasoning see the very end of my answer.
2. Compute $mathbb{E}(X_t)$: Since $mathbb{E}(B_s)=0$ for all $s geq 0$, it follows that each $X_t^{(n)}$ (defined in $(1)$) has expectation zero, and hence its limit $X_t = lim_n X_t^{(n)}$ has expectation zero. Alternatively, we can apply Fubini's theorem:
$$mathbb{E}(X_t) = mathbb{E} left( int_0^t B_s , ds right) = int_0^t underbrace{mathbb{E}(B_s)}_{=0} , ds =0.$$
3. Compute $text{var}(X_t)$: Since we already know that $mathbb{E}(X_t)=0$, we clearly have $text{var}(X_t) = mathbb{E}(X_t^2)$. As
$$X_t^2 = int_0^t int_0^t B_s B_r , ds , dr$$
it follows from Fubini's theorem that
$$mathbb{E}(X_t^2) = int_0^t int_0^t mathbb{E}(B_s B_r) , ds , dr. tag{2}$$
By symmetry, we thus get
$$mathbb{E}(X_t^2) = 2 int_0^t int_0^r underbrace{mathbb{E}(B_s B_r)}_{min{s,r}=s} , ds , dr = 2 int_0^t int_0^r s , ds = frac{t^3}{3}$$
If you don't like symmetrization, then note that (2) implies
$$mathbb{E}(X_t^2) = int_0^t int_0^s underbrace{mathbb{E}(B_s B_r)}_{min{s,r}=s} , ds , dr + int_0^t int_s^t underbrace{mathbb{E}(B_s B_r)}_{min{s,r}=r} , ds , dr$$
and each of the integrals can be calculated explicitly using standard calculus.
Let me close this answer with a result which combines all the three steps into one.
Proposition: Let $(L_t)_{t geq 0}$ be a Lévy process with characteristic exponent $psi$, i.e. $$mathbb{E}exp(i xi L_t) = exp(-t psi(xi)), qquad t geq 0, xi in mathbb{R}. tag{3}$$ Then the characteristic function of $$X_t := int_0^t L_s , ds$$ equals $$phi(xi) = exp left(- int_0^t psi(xi s) , ds right), qquad xi in mathbb{R}.$$
If you are not familar with Lévy processes (that it, stochastic processes with independent and stationary increments), then you can just think of a Brownian motion; in this case $psi$ is given by $psi(xi) = xi^2/2$. Applying the proposition, we thus find that the characteristic function of $X_t = int_0^t B_s , ds$ equals $$exp left(- frac{t^3}{3} frac{xi^2}{2} right)$$ which is the characteristic function of $N(0,t^3/3)$, and so $X_t sim N(0,t^3/3)$.
Proof of the proposition: For fixed $n in mathbb{N}$ and $t>0$ set $t_j := t j/n$ for $j=1,ldots,n$, and set
$$phi_n(xi) := mathbb{E} exp left( i xi frac{1}{n} sum_{j=1}^n L_{t_j} right).$$
Denote by $mathcal{F}_t := sigma(L_s; s leq t)$ the canonical filtration of $(L_t)_{t geq 0}$. Using the tower property of the conditional expectation, we find
$$begin{align*} phi_n(xi) &= mathbb{E} bigg{ mathbb{E} bigg[ exp left( i xi frac{1}{n} sum_{j=1}^n L_{t_j} right) mid mathcal{F}_{t_{n-1}} bigg] bigg} \ &= mathbb{E} bigg{ exp left( i xi frac{1}{n} sum_{j=1}^{n-1} L_{t_j} right) mathbb{E} bigg[ exp left( i xi frac{1}{n} L_{t_n} right) mid mathcal{F}_{t_{n-1}} bigg] bigg} tag{4} end{align*}$$
Since $(L_t)_{t geq 0}$ has independent increments, we have
$$begin{align*} mathbb{E} bigg[ exp left( i xi frac{1}{n} L_{t_n} right) mid mathcal{F}_{t_{n-1}} bigg] &=exp(i xi/n L_{t_{n-1}}) mathbb{E} bigg[ exp left( i xi frac{1}{n} (L_{t_n}-L_{t_{n-1}}) right) mid mathcal{F}_{t_{n-1}} bigg] \ &= exp(i xi/n L_{t_{n-1}}) mathbb{E}expleft( i xi frac{1}{n} (L_{t_n}-L_{t_{n-1}}) right). end{align*}$$
Using that $(L_t)_{t geq 0}$ has stationary increments, i.e. $L_{t_n}-L_{t_{n-1}} sim L_{t_n-t_{n-1}}=L_{1/n}$, and using $(3)$ we thus get
$$ mathbb{E} bigg[ exp left( i xi frac{1}{n} L_{t_n} right) mid mathcal{F}_{t_{n-1}} bigg] = exp(i xi/n L_{t_{n-1}}) exp left(- frac{1}{n} psi left( frac{xi}{n} right) right).$$
Plugging this into $(4)$, we obtain that
$$phi_n(xi) = mathbb{E} bigg{ exp left( i xi frac{1}{n} sum_{j=1}^{n-2} L_{t_j} + i xi frac{2}{n} L_{t_{n-1}} right) exp left(- frac{1}{n} psi left( frac{xi}{n} right) right) .$$
Iterating this reasoning (i.e. next conditioning on $mathcal{F}_{t_{n-2}}$, then on $mathcal{F}_{t_{n-3}}$, ...) we conclude that
$$phi_n(xi) = exp left( - frac{1}{n} sum_{j=1}^n psi left( frac{j}{n} xi right) right).tag{5}$$
Finally, we note that
$$X_t stackrel{text{def}}{=} int_0^t L_s , ds = lim_{n to infty} frac{1}{n} sum_{j=1}^n L_{tj/n},$$
and thus, by letting $n to infty$ in (5), we get
$$mathbb{E}exp(i xi X_t) = exp left(- int_0^t psi(s xi) , ds right).$$
$endgroup$
There are three things which need to be done:
- Check that $X_t$ is Gaussian.
- Compute its expectation.
- Compute its variance.
1. $X_t$ is Gaussian: It seems to me that the most natural (and also direct) way to prove this is an approximation by Riemann sums. Noting that
$$X_t^{(n)} := sum_{j=1}^n frac{1}{n} B_{t j/n} tag{1}$$
is Gaussian for each $t>0$ (because $(B_t)_{t geq 0}$ is a Gaussian process), we find that $$X_t = lim_{n to infty} X_t^{(n)}$$ is Gaussian as pointwise limit of Gaussian random variables. For an alternative reasoning see the very end of my answer.
2. Compute $mathbb{E}(X_t)$: Since $mathbb{E}(B_s)=0$ for all $s geq 0$, it follows that each $X_t^{(n)}$ (defined in $(1)$) has expectation zero, and hence its limit $X_t = lim_n X_t^{(n)}$ has expectation zero. Alternatively, we can apply Fubini's theorem:
$$mathbb{E}(X_t) = mathbb{E} left( int_0^t B_s , ds right) = int_0^t underbrace{mathbb{E}(B_s)}_{=0} , ds =0.$$
3. Compute $text{var}(X_t)$: Since we already know that $mathbb{E}(X_t)=0$, we clearly have $text{var}(X_t) = mathbb{E}(X_t^2)$. As
$$X_t^2 = int_0^t int_0^t B_s B_r , ds , dr$$
it follows from Fubini's theorem that
$$mathbb{E}(X_t^2) = int_0^t int_0^t mathbb{E}(B_s B_r) , ds , dr. tag{2}$$
By symmetry, we thus get
$$mathbb{E}(X_t^2) = 2 int_0^t int_0^r underbrace{mathbb{E}(B_s B_r)}_{min{s,r}=s} , ds , dr = 2 int_0^t int_0^r s , ds = frac{t^3}{3}$$
If you don't like symmetrization, then note that (2) implies
$$mathbb{E}(X_t^2) = int_0^t int_0^s underbrace{mathbb{E}(B_s B_r)}_{min{s,r}=s} , ds , dr + int_0^t int_s^t underbrace{mathbb{E}(B_s B_r)}_{min{s,r}=r} , ds , dr$$
and each of the integrals can be calculated explicitly using standard calculus.
Let me close this answer with a result which combines all the three steps into one.
Proposition: Let $(L_t)_{t geq 0}$ be a Lévy process with characteristic exponent $psi$, i.e. $$mathbb{E}exp(i xi L_t) = exp(-t psi(xi)), qquad t geq 0, xi in mathbb{R}. tag{3}$$ Then the characteristic function of $$X_t := int_0^t L_s , ds$$ equals $$phi(xi) = exp left(- int_0^t psi(xi s) , ds right), qquad xi in mathbb{R}.$$
If you are not familar with Lévy processes (that it, stochastic processes with independent and stationary increments), then you can just think of a Brownian motion; in this case $psi$ is given by $psi(xi) = xi^2/2$. Applying the proposition, we thus find that the characteristic function of $X_t = int_0^t B_s , ds$ equals $$exp left(- frac{t^3}{3} frac{xi^2}{2} right)$$ which is the characteristic function of $N(0,t^3/3)$, and so $X_t sim N(0,t^3/3)$.
Proof of the proposition: For fixed $n in mathbb{N}$ and $t>0$ set $t_j := t j/n$ for $j=1,ldots,n$, and set
$$phi_n(xi) := mathbb{E} exp left( i xi frac{1}{n} sum_{j=1}^n L_{t_j} right).$$
Denote by $mathcal{F}_t := sigma(L_s; s leq t)$ the canonical filtration of $(L_t)_{t geq 0}$. Using the tower property of the conditional expectation, we find
$$begin{align*} phi_n(xi) &= mathbb{E} bigg{ mathbb{E} bigg[ exp left( i xi frac{1}{n} sum_{j=1}^n L_{t_j} right) mid mathcal{F}_{t_{n-1}} bigg] bigg} \ &= mathbb{E} bigg{ exp left( i xi frac{1}{n} sum_{j=1}^{n-1} L_{t_j} right) mathbb{E} bigg[ exp left( i xi frac{1}{n} L_{t_n} right) mid mathcal{F}_{t_{n-1}} bigg] bigg} tag{4} end{align*}$$
Since $(L_t)_{t geq 0}$ has independent increments, we have
$$begin{align*} mathbb{E} bigg[ exp left( i xi frac{1}{n} L_{t_n} right) mid mathcal{F}_{t_{n-1}} bigg] &=exp(i xi/n L_{t_{n-1}}) mathbb{E} bigg[ exp left( i xi frac{1}{n} (L_{t_n}-L_{t_{n-1}}) right) mid mathcal{F}_{t_{n-1}} bigg] \ &= exp(i xi/n L_{t_{n-1}}) mathbb{E}expleft( i xi frac{1}{n} (L_{t_n}-L_{t_{n-1}}) right). end{align*}$$
Using that $(L_t)_{t geq 0}$ has stationary increments, i.e. $L_{t_n}-L_{t_{n-1}} sim L_{t_n-t_{n-1}}=L_{1/n}$, and using $(3)$ we thus get
$$ mathbb{E} bigg[ exp left( i xi frac{1}{n} L_{t_n} right) mid mathcal{F}_{t_{n-1}} bigg] = exp(i xi/n L_{t_{n-1}}) exp left(- frac{1}{n} psi left( frac{xi}{n} right) right).$$
Plugging this into $(4)$, we obtain that
$$phi_n(xi) = mathbb{E} bigg{ exp left( i xi frac{1}{n} sum_{j=1}^{n-2} L_{t_j} + i xi frac{2}{n} L_{t_{n-1}} right) exp left(- frac{1}{n} psi left( frac{xi}{n} right) right) .$$
Iterating this reasoning (i.e. next conditioning on $mathcal{F}_{t_{n-2}}$, then on $mathcal{F}_{t_{n-3}}$, ...) we conclude that
$$phi_n(xi) = exp left( - frac{1}{n} sum_{j=1}^n psi left( frac{j}{n} xi right) right).tag{5}$$
Finally, we note that
$$X_t stackrel{text{def}}{=} int_0^t L_s , ds = lim_{n to infty} frac{1}{n} sum_{j=1}^n L_{tj/n},$$
and thus, by letting $n to infty$ in (5), we get
$$mathbb{E}exp(i xi X_t) = exp left(- int_0^t psi(s xi) , ds right).$$
edited Jan 17 at 15:05
answered Jan 16 at 18:19
sazsaz
80.4k860125
80.4k860125
$begingroup$
Thanks for such a detailed answer!
$endgroup$
– Vim
Jan 17 at 0:39
$begingroup$
@Vim You're welcome.
$endgroup$
– saz
Jan 17 at 7:30
add a comment |
$begingroup$
Thanks for such a detailed answer!
$endgroup$
– Vim
Jan 17 at 0:39
$begingroup$
@Vim You're welcome.
$endgroup$
– saz
Jan 17 at 7:30
$begingroup$
Thanks for such a detailed answer!
$endgroup$
– Vim
Jan 17 at 0:39
$begingroup$
Thanks for such a detailed answer!
$endgroup$
– Vim
Jan 17 at 0:39
$begingroup$
@Vim You're welcome.
$endgroup$
– saz
Jan 17 at 7:30
$begingroup$
@Vim You're welcome.
$endgroup$
– saz
Jan 17 at 7:30
add a comment |
Thanks for contributing an answer to Mathematics Stack Exchange!
- Please be sure to answer the question. Provide details and share your research!
But avoid …
- Asking for help, clarification, or responding to other answers.
- Making statements based on opinion; back them up with references or personal experience.
Use MathJax to format equations. MathJax reference.
To learn more, see our tips on writing great answers.
Sign up or log in
StackExchange.ready(function () {
StackExchange.helpers.onClickDraftSave('#login-link');
});
Sign up using Google
Sign up using Facebook
Sign up using Email and Password
Post as a guest
Required, but never shown
StackExchange.ready(
function () {
StackExchange.openid.initPostLogin('.new-post-login', 'https%3a%2f%2fmath.stackexchange.com%2fquestions%2f3075464%2fhow-to-easily-see-the-time-integral-of-a-brownian-motion-is-normally-distributed%23new-answer', 'question_page');
}
);
Post as a guest
Required, but never shown
Sign up or log in
StackExchange.ready(function () {
StackExchange.helpers.onClickDraftSave('#login-link');
});
Sign up using Google
Sign up using Facebook
Sign up using Email and Password
Post as a guest
Required, but never shown
Sign up or log in
StackExchange.ready(function () {
StackExchange.helpers.onClickDraftSave('#login-link');
});
Sign up using Google
Sign up using Facebook
Sign up using Email and Password
Post as a guest
Required, but never shown
Sign up or log in
StackExchange.ready(function () {
StackExchange.helpers.onClickDraftSave('#login-link');
});
Sign up using Google
Sign up using Facebook
Sign up using Email and Password
Sign up using Google
Sign up using Facebook
Sign up using Email and Password
Post as a guest
Required, but never shown
Required, but never shown
Required, but never shown
Required, but never shown
Required, but never shown
Required, but never shown
Required, but never shown
Required, but never shown
Required, but never shown
bFpN1UO4akqXEUahsQvxnJ 5U6,zj2RXY5Hbbj
1
$begingroup$
Writing the integral as limit of Riemann sums is ths natural easiest way to prove this.
$endgroup$
– Kavi Rama Murthy
Jan 16 at 8:26
$begingroup$
@KaviRamaMurthy Ok, but I'm still expecting something easier, like a "continuous" argument (cleverly determine its characterisitc function, so to speak). Discretisation looks non-tidy, albeit natural.
$endgroup$
– Vim
Jan 16 at 8:29
$begingroup$
Why do you have to breat $B_{tau_i}$ into tiny intervals...? The random vector $(B_{t_1},ldots,B_{t_n})$ is Gaussian for any choice of $t_i$ (as $B$ is a Gaussian process), and hence $$sum_{i=1}^n c_i B_{t_i}$$ is Gaussian for any choice of constants $c_i$.
$endgroup$
– saz
Jan 16 at 8:57
$begingroup$
@saz you're definitely correct. It's just that estimating the variance of independent sums is easier.
$endgroup$
– Vim
Jan 16 at 12:53
1
$begingroup$
@Vim Well, the expectation as well as the variance can be calculated using Fubini's theorem... that's not really a problem. From my point of view, the key point is to show that $X_t$ is Gaussian. By the way, I don't think that it possible to get rid of the discretisation - simply because the integral is defined as the limit of the discretisations. Obviously, you can apply some "big" result and hide the discretisation there... but that doesn't make the proof "easier", in my oppinion.
$endgroup$
– saz
Jan 16 at 14:03