Conditional variance when there is uncertainity about the distribution of condition
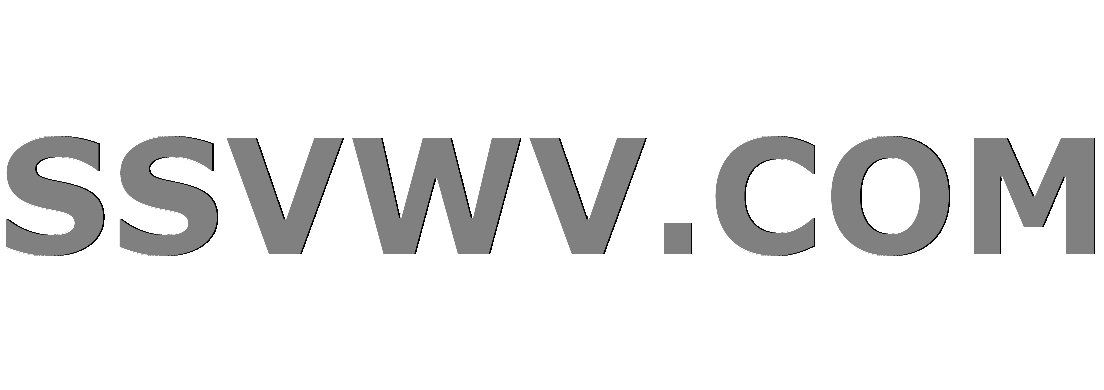
Multi tool use
$begingroup$
Assume that $X sim N(0,sigma_x^2)$. Y has the following form
begin{align}
Y &=
begin{cases}
Y_1 sim N(0, sigma_1^2), & text{w.p.} quad mu \
Y_2 sim N(0, sigma_2^2), & text{w.p.} quad (1-mu)
end{cases}
end{align}
where $Cov(X,Y_1) =Sigma_1$, and $Cov(X,Y_2) =Sigma_2$. And also X and Y1, and X and Y2 are both bivariate normally distributed.
How can we calculate $V(X|Y=y)$?
Is the following idea correct?
begin{align*}
V(X|Y=y) = mu V(X|Y=y_1)+(1-mu)V(X|Y=y_2)
end{align*}
Basically, y is realized but it might be in the from of $y_1 in Y_1$ or $y_2 in Y_2$. What do you think?
normal-distribution conditional-expectation conditional-probability variance
$endgroup$
add a comment |
$begingroup$
Assume that $X sim N(0,sigma_x^2)$. Y has the following form
begin{align}
Y &=
begin{cases}
Y_1 sim N(0, sigma_1^2), & text{w.p.} quad mu \
Y_2 sim N(0, sigma_2^2), & text{w.p.} quad (1-mu)
end{cases}
end{align}
where $Cov(X,Y_1) =Sigma_1$, and $Cov(X,Y_2) =Sigma_2$. And also X and Y1, and X and Y2 are both bivariate normally distributed.
How can we calculate $V(X|Y=y)$?
Is the following idea correct?
begin{align*}
V(X|Y=y) = mu V(X|Y=y_1)+(1-mu)V(X|Y=y_2)
end{align*}
Basically, y is realized but it might be in the from of $y_1 in Y_1$ or $y_2 in Y_2$. What do you think?
normal-distribution conditional-expectation conditional-probability variance
$endgroup$
$begingroup$
I think we need more information to solve this problem: what is the joint distribution of $X$ and $Y_1$ (and $X$ with $Y_2$)? We need to know the distribution of $X$ given $Y = y, Y =Y_1$ (and $Y = y, Y =Y_2$) in order to solve this question. Can we assume that it is a bivariate normal distribution? From the information that you've given so far we can't: this post gives an example where $X, Y$ are both normal and have non-zero covariance but are not bivariate normal stats.stackexchange.com/questions/293818/…
$endgroup$
– Alex
Jan 22 at 8:14
$begingroup$
$X$ and $Y_1$, and $X$ and $Y_2$ are both bivariate normal distribution. But when $y$ is realized it is not known whether it comes from $Y_1$ or $Y_2$. Is this information enough, @Alex.
$endgroup$
– user229519
Jan 22 at 14:14
add a comment |
$begingroup$
Assume that $X sim N(0,sigma_x^2)$. Y has the following form
begin{align}
Y &=
begin{cases}
Y_1 sim N(0, sigma_1^2), & text{w.p.} quad mu \
Y_2 sim N(0, sigma_2^2), & text{w.p.} quad (1-mu)
end{cases}
end{align}
where $Cov(X,Y_1) =Sigma_1$, and $Cov(X,Y_2) =Sigma_2$. And also X and Y1, and X and Y2 are both bivariate normally distributed.
How can we calculate $V(X|Y=y)$?
Is the following idea correct?
begin{align*}
V(X|Y=y) = mu V(X|Y=y_1)+(1-mu)V(X|Y=y_2)
end{align*}
Basically, y is realized but it might be in the from of $y_1 in Y_1$ or $y_2 in Y_2$. What do you think?
normal-distribution conditional-expectation conditional-probability variance
$endgroup$
Assume that $X sim N(0,sigma_x^2)$. Y has the following form
begin{align}
Y &=
begin{cases}
Y_1 sim N(0, sigma_1^2), & text{w.p.} quad mu \
Y_2 sim N(0, sigma_2^2), & text{w.p.} quad (1-mu)
end{cases}
end{align}
where $Cov(X,Y_1) =Sigma_1$, and $Cov(X,Y_2) =Sigma_2$. And also X and Y1, and X and Y2 are both bivariate normally distributed.
How can we calculate $V(X|Y=y)$?
Is the following idea correct?
begin{align*}
V(X|Y=y) = mu V(X|Y=y_1)+(1-mu)V(X|Y=y_2)
end{align*}
Basically, y is realized but it might be in the from of $y_1 in Y_1$ or $y_2 in Y_2$. What do you think?
normal-distribution conditional-expectation conditional-probability variance
normal-distribution conditional-expectation conditional-probability variance
edited Jan 22 at 14:22
user229519
asked Jan 21 at 22:15
user229519user229519
105
105
$begingroup$
I think we need more information to solve this problem: what is the joint distribution of $X$ and $Y_1$ (and $X$ with $Y_2$)? We need to know the distribution of $X$ given $Y = y, Y =Y_1$ (and $Y = y, Y =Y_2$) in order to solve this question. Can we assume that it is a bivariate normal distribution? From the information that you've given so far we can't: this post gives an example where $X, Y$ are both normal and have non-zero covariance but are not bivariate normal stats.stackexchange.com/questions/293818/…
$endgroup$
– Alex
Jan 22 at 8:14
$begingroup$
$X$ and $Y_1$, and $X$ and $Y_2$ are both bivariate normal distribution. But when $y$ is realized it is not known whether it comes from $Y_1$ or $Y_2$. Is this information enough, @Alex.
$endgroup$
– user229519
Jan 22 at 14:14
add a comment |
$begingroup$
I think we need more information to solve this problem: what is the joint distribution of $X$ and $Y_1$ (and $X$ with $Y_2$)? We need to know the distribution of $X$ given $Y = y, Y =Y_1$ (and $Y = y, Y =Y_2$) in order to solve this question. Can we assume that it is a bivariate normal distribution? From the information that you've given so far we can't: this post gives an example where $X, Y$ are both normal and have non-zero covariance but are not bivariate normal stats.stackexchange.com/questions/293818/…
$endgroup$
– Alex
Jan 22 at 8:14
$begingroup$
$X$ and $Y_1$, and $X$ and $Y_2$ are both bivariate normal distribution. But when $y$ is realized it is not known whether it comes from $Y_1$ or $Y_2$. Is this information enough, @Alex.
$endgroup$
– user229519
Jan 22 at 14:14
$begingroup$
I think we need more information to solve this problem: what is the joint distribution of $X$ and $Y_1$ (and $X$ with $Y_2$)? We need to know the distribution of $X$ given $Y = y, Y =Y_1$ (and $Y = y, Y =Y_2$) in order to solve this question. Can we assume that it is a bivariate normal distribution? From the information that you've given so far we can't: this post gives an example where $X, Y$ are both normal and have non-zero covariance but are not bivariate normal stats.stackexchange.com/questions/293818/…
$endgroup$
– Alex
Jan 22 at 8:14
$begingroup$
I think we need more information to solve this problem: what is the joint distribution of $X$ and $Y_1$ (and $X$ with $Y_2$)? We need to know the distribution of $X$ given $Y = y, Y =Y_1$ (and $Y = y, Y =Y_2$) in order to solve this question. Can we assume that it is a bivariate normal distribution? From the information that you've given so far we can't: this post gives an example where $X, Y$ are both normal and have non-zero covariance but are not bivariate normal stats.stackexchange.com/questions/293818/…
$endgroup$
– Alex
Jan 22 at 8:14
$begingroup$
$X$ and $Y_1$, and $X$ and $Y_2$ are both bivariate normal distribution. But when $y$ is realized it is not known whether it comes from $Y_1$ or $Y_2$. Is this information enough, @Alex.
$endgroup$
– user229519
Jan 22 at 14:14
$begingroup$
$X$ and $Y_1$, and $X$ and $Y_2$ are both bivariate normal distribution. But when $y$ is realized it is not known whether it comes from $Y_1$ or $Y_2$. Is this information enough, @Alex.
$endgroup$
– user229519
Jan 22 at 14:14
add a comment |
1 Answer
1
active
oldest
votes
$begingroup$
You can use the Conditional Variance Formula:
$$mathbb{V}(X mid Y = y) = mathbb{E}((X - mathbb{E}(Xmid Y = y))^{2}mid Y = y)$$
We need to calculate $mathbb{E}(X mid Y = y)$, and we can do this using a variation of the Law of Total Expectation. Let $A_i$ be the event $Y = Y_i$.
$$mathbb{E}(X mid Y = y) = int xthinspace f_{x mid y}dx = int xfrac{f_{x,y}}{f_y}dx = int xsum_i frac{f_{x,y,A_i}}{f_y}dx$$
$$= int xsum_i frac{f_{x,y,A_i}}{f_{y,A_i}}frac{f_{y,A_i}}{f_{y}}dx= int xsum_i frac{f_{x,y,A_i}}{f_{y,A_i}}frac{f_{y,A_i}}{f_{y}}dx = sum_iint x thinspace f_{x mid y, A_i}mathbb{P}(A_i mid Y = y)dx$$
$$= sum_i int x thinspace f_{x mid y, A_i}dx cdot mathbb{P}(A_i mid Y = y) = sum_i mathbb{E}(X mid Y = y, A_i)mathbb{P}(A_i mid Y = y).$$
So
$$mathbb{E}(X mid Y = y) = mathbb{E}(X mid Y = y, Y = Y_1) mathbb{P}(Y = Y_1 mid Y = y) + mathbb{E}(X mid Y = y, Y = Y_2) mathbb{P}(Y = Y_2 mid Y = y).$$
We can use Bayes' Theorem to calculate the probabilities above:
$$mathbb{P}(Y = Y_1 mid Y = y) = frac{f_{Y_1}(y) mathbb{P}(Y = Y_1)}{f_{Y_1}(y) mathbb{P}(Y = Y_1) + f_{Y_2}(y) mathbb{P}(Y = Y_2)}$$
where
$$f_{Y_i}(y) = frac{1}{sqrt{2pi sigma_i^2}}e^{-y^2/2sigma_i^2} text{for } i = 1, 2.$$
So
$$mathbb{P}(Y = Y_1 mid Y = y) = frac{1/sigma_1 e^{-y^2/2sigma_1^2} mu}{1/sigma_1 e^{-y^2/2sigma_1^2} mu + 1/sigma_2 e^{-y^2/2sigma_2^2} (1 -mu)} = frac{sigma_2 mu e^{-y^2/2sigma_1^2}}{sigma_2 mu e^{-y^2/2sigma_1^2} + sigma_1 (1 - mu)e^{-y^2/2sigma_2^2}}.$$
Also
$$mathbb{P}(Y = Y_2 mid Y = y) = frac{sigma_1 (1 - mu) e^{-y^2/2sigma_2^2}}{sigma_2 mu e^{-y^2/2sigma_1^2} + sigma_1 (1 - mu)e^{-y^2/2sigma_2^2}}.$$
The conditional distribution of $X mid Y = y, Y = Y_i$ is given by
$$X mid Y = y, Y = Y_i sim Nleft(mu_x + rho_ifrac{sigma_x}{sigma_i}(y - mu_i), sigma_x^2 (1 - rho_i^2) right)$$
where $rho_i$ is the correlation of $X$ and $Y_i$, $mu_x = mathbb{E}(X) = 0$ and $mu_i = mathbb{E}(Y_i) = 0$.
Putting this together we have
$$mathbb{E}(X mid Y = y) = rho_1frac{sigma_x}{sigma_1}y cdot p_1 + rho_2frac{sigma_x}{sigma_2}y cdot p_2 = mu_{x mid y},$$
where $p_i = mathbb{P}(Y = Y_i mid Y = y)$.
Returning to the conditional variance formula, we now expand it, conditioning on $Y = Y_i$ again:
$$mathbb{V}(X mid Y = y) = mathbb{E}((X - mu_{xmid y})^{2}mid Y = y)$$
$$= mathbb{E}((X - mu_{xmid y})^{2}mid Y = y, Y = Y_1)mathbb{P}(Y = Y_1 mid Y = y) + mathbb{E}((X - mu_{xmid y})^{2}mid Y = y, Y = Y_2)mathbb{P}(Y = Y_2 mid Y = y).$$
Now $$(X - mu_{xmid y})^{2} = (X - mu_{x mid y,i} + mu_{x mid y,i} - mu_{xmid y})^{2} = (X - mu_{x mid y,i})^2 + 2(X - mu_{x mid y,i})(mu_{x mid y,i} - mu_{xmid y}) + (mu_{x mid y,i} - mu_{xmid y})^2,$$
where $mu_{x mid y,i} = mathbb{E}(X mid Y = y, Y = Y_i)$, so
$$mathbb{E}((X - mu_{xmid y})^{2}mid Y = y = Y_i) = mathbb{E}((X - mu_{x mid y,i})^2 + 2(X - mu_{x mid y,i})(mu_{x mid y,i} - mu_{xmid y}) + (mu_{x mid y,i} - mu_{xmid y})^2mid Y = y = Y_i)$$
$$= sigma_x^2 (1 - rho_i^2) + 0 + (mu_{x mid y,i} - mu_{xmid y})^2.$$
Therefore
$$mathbb{V}(X mid Y = y) = left(sigma_x^2 (1 - rho_1^2) + (mu_{x mid y,1} - mu_{xmid y})^2 right)p_1 + left(sigma_x^2 (1 - rho_2^2) + (mu_{x mid y,2} - mu_{xmid y})^2 right)p_2.$$
$$ = sigma_x^2 + left(- sigma_x^2 rho_1^2 + (mu_{x mid y,1} - mu_{xmid y})^2 right)p_1 + left(- sigma_x^2 rho_2^2 + (mu_{x mid y,2} - mu_{xmid y})^2 right)p_2.$$
$endgroup$
1
$begingroup$
Thanks for your effort, Alex, really appreciate that.
$endgroup$
– user229519
Jan 23 at 20:31
add a comment |
Your Answer
StackExchange.ifUsing("editor", function () {
return StackExchange.using("mathjaxEditing", function () {
StackExchange.MarkdownEditor.creationCallbacks.add(function (editor, postfix) {
StackExchange.mathjaxEditing.prepareWmdForMathJax(editor, postfix, [["$", "$"], ["\\(","\\)"]]);
});
});
}, "mathjax-editing");
StackExchange.ready(function() {
var channelOptions = {
tags: "".split(" "),
id: "69"
};
initTagRenderer("".split(" "), "".split(" "), channelOptions);
StackExchange.using("externalEditor", function() {
// Have to fire editor after snippets, if snippets enabled
if (StackExchange.settings.snippets.snippetsEnabled) {
StackExchange.using("snippets", function() {
createEditor();
});
}
else {
createEditor();
}
});
function createEditor() {
StackExchange.prepareEditor({
heartbeatType: 'answer',
autoActivateHeartbeat: false,
convertImagesToLinks: true,
noModals: true,
showLowRepImageUploadWarning: true,
reputationToPostImages: 10,
bindNavPrevention: true,
postfix: "",
imageUploader: {
brandingHtml: "Powered by u003ca class="icon-imgur-white" href="https://imgur.com/"u003eu003c/au003e",
contentPolicyHtml: "User contributions licensed under u003ca href="https://creativecommons.org/licenses/by-sa/3.0/"u003ecc by-sa 3.0 with attribution requiredu003c/au003e u003ca href="https://stackoverflow.com/legal/content-policy"u003e(content policy)u003c/au003e",
allowUrls: true
},
noCode: true, onDemand: true,
discardSelector: ".discard-answer"
,immediatelyShowMarkdownHelp:true
});
}
});
Sign up or log in
StackExchange.ready(function () {
StackExchange.helpers.onClickDraftSave('#login-link');
});
Sign up using Google
Sign up using Facebook
Sign up using Email and Password
Post as a guest
Required, but never shown
StackExchange.ready(
function () {
StackExchange.openid.initPostLogin('.new-post-login', 'https%3a%2f%2fmath.stackexchange.com%2fquestions%2f3082490%2fconditional-variance-when-there-is-uncertainity-about-the-distribution-of-condit%23new-answer', 'question_page');
}
);
Post as a guest
Required, but never shown
1 Answer
1
active
oldest
votes
1 Answer
1
active
oldest
votes
active
oldest
votes
active
oldest
votes
$begingroup$
You can use the Conditional Variance Formula:
$$mathbb{V}(X mid Y = y) = mathbb{E}((X - mathbb{E}(Xmid Y = y))^{2}mid Y = y)$$
We need to calculate $mathbb{E}(X mid Y = y)$, and we can do this using a variation of the Law of Total Expectation. Let $A_i$ be the event $Y = Y_i$.
$$mathbb{E}(X mid Y = y) = int xthinspace f_{x mid y}dx = int xfrac{f_{x,y}}{f_y}dx = int xsum_i frac{f_{x,y,A_i}}{f_y}dx$$
$$= int xsum_i frac{f_{x,y,A_i}}{f_{y,A_i}}frac{f_{y,A_i}}{f_{y}}dx= int xsum_i frac{f_{x,y,A_i}}{f_{y,A_i}}frac{f_{y,A_i}}{f_{y}}dx = sum_iint x thinspace f_{x mid y, A_i}mathbb{P}(A_i mid Y = y)dx$$
$$= sum_i int x thinspace f_{x mid y, A_i}dx cdot mathbb{P}(A_i mid Y = y) = sum_i mathbb{E}(X mid Y = y, A_i)mathbb{P}(A_i mid Y = y).$$
So
$$mathbb{E}(X mid Y = y) = mathbb{E}(X mid Y = y, Y = Y_1) mathbb{P}(Y = Y_1 mid Y = y) + mathbb{E}(X mid Y = y, Y = Y_2) mathbb{P}(Y = Y_2 mid Y = y).$$
We can use Bayes' Theorem to calculate the probabilities above:
$$mathbb{P}(Y = Y_1 mid Y = y) = frac{f_{Y_1}(y) mathbb{P}(Y = Y_1)}{f_{Y_1}(y) mathbb{P}(Y = Y_1) + f_{Y_2}(y) mathbb{P}(Y = Y_2)}$$
where
$$f_{Y_i}(y) = frac{1}{sqrt{2pi sigma_i^2}}e^{-y^2/2sigma_i^2} text{for } i = 1, 2.$$
So
$$mathbb{P}(Y = Y_1 mid Y = y) = frac{1/sigma_1 e^{-y^2/2sigma_1^2} mu}{1/sigma_1 e^{-y^2/2sigma_1^2} mu + 1/sigma_2 e^{-y^2/2sigma_2^2} (1 -mu)} = frac{sigma_2 mu e^{-y^2/2sigma_1^2}}{sigma_2 mu e^{-y^2/2sigma_1^2} + sigma_1 (1 - mu)e^{-y^2/2sigma_2^2}}.$$
Also
$$mathbb{P}(Y = Y_2 mid Y = y) = frac{sigma_1 (1 - mu) e^{-y^2/2sigma_2^2}}{sigma_2 mu e^{-y^2/2sigma_1^2} + sigma_1 (1 - mu)e^{-y^2/2sigma_2^2}}.$$
The conditional distribution of $X mid Y = y, Y = Y_i$ is given by
$$X mid Y = y, Y = Y_i sim Nleft(mu_x + rho_ifrac{sigma_x}{sigma_i}(y - mu_i), sigma_x^2 (1 - rho_i^2) right)$$
where $rho_i$ is the correlation of $X$ and $Y_i$, $mu_x = mathbb{E}(X) = 0$ and $mu_i = mathbb{E}(Y_i) = 0$.
Putting this together we have
$$mathbb{E}(X mid Y = y) = rho_1frac{sigma_x}{sigma_1}y cdot p_1 + rho_2frac{sigma_x}{sigma_2}y cdot p_2 = mu_{x mid y},$$
where $p_i = mathbb{P}(Y = Y_i mid Y = y)$.
Returning to the conditional variance formula, we now expand it, conditioning on $Y = Y_i$ again:
$$mathbb{V}(X mid Y = y) = mathbb{E}((X - mu_{xmid y})^{2}mid Y = y)$$
$$= mathbb{E}((X - mu_{xmid y})^{2}mid Y = y, Y = Y_1)mathbb{P}(Y = Y_1 mid Y = y) + mathbb{E}((X - mu_{xmid y})^{2}mid Y = y, Y = Y_2)mathbb{P}(Y = Y_2 mid Y = y).$$
Now $$(X - mu_{xmid y})^{2} = (X - mu_{x mid y,i} + mu_{x mid y,i} - mu_{xmid y})^{2} = (X - mu_{x mid y,i})^2 + 2(X - mu_{x mid y,i})(mu_{x mid y,i} - mu_{xmid y}) + (mu_{x mid y,i} - mu_{xmid y})^2,$$
where $mu_{x mid y,i} = mathbb{E}(X mid Y = y, Y = Y_i)$, so
$$mathbb{E}((X - mu_{xmid y})^{2}mid Y = y = Y_i) = mathbb{E}((X - mu_{x mid y,i})^2 + 2(X - mu_{x mid y,i})(mu_{x mid y,i} - mu_{xmid y}) + (mu_{x mid y,i} - mu_{xmid y})^2mid Y = y = Y_i)$$
$$= sigma_x^2 (1 - rho_i^2) + 0 + (mu_{x mid y,i} - mu_{xmid y})^2.$$
Therefore
$$mathbb{V}(X mid Y = y) = left(sigma_x^2 (1 - rho_1^2) + (mu_{x mid y,1} - mu_{xmid y})^2 right)p_1 + left(sigma_x^2 (1 - rho_2^2) + (mu_{x mid y,2} - mu_{xmid y})^2 right)p_2.$$
$$ = sigma_x^2 + left(- sigma_x^2 rho_1^2 + (mu_{x mid y,1} - mu_{xmid y})^2 right)p_1 + left(- sigma_x^2 rho_2^2 + (mu_{x mid y,2} - mu_{xmid y})^2 right)p_2.$$
$endgroup$
1
$begingroup$
Thanks for your effort, Alex, really appreciate that.
$endgroup$
– user229519
Jan 23 at 20:31
add a comment |
$begingroup$
You can use the Conditional Variance Formula:
$$mathbb{V}(X mid Y = y) = mathbb{E}((X - mathbb{E}(Xmid Y = y))^{2}mid Y = y)$$
We need to calculate $mathbb{E}(X mid Y = y)$, and we can do this using a variation of the Law of Total Expectation. Let $A_i$ be the event $Y = Y_i$.
$$mathbb{E}(X mid Y = y) = int xthinspace f_{x mid y}dx = int xfrac{f_{x,y}}{f_y}dx = int xsum_i frac{f_{x,y,A_i}}{f_y}dx$$
$$= int xsum_i frac{f_{x,y,A_i}}{f_{y,A_i}}frac{f_{y,A_i}}{f_{y}}dx= int xsum_i frac{f_{x,y,A_i}}{f_{y,A_i}}frac{f_{y,A_i}}{f_{y}}dx = sum_iint x thinspace f_{x mid y, A_i}mathbb{P}(A_i mid Y = y)dx$$
$$= sum_i int x thinspace f_{x mid y, A_i}dx cdot mathbb{P}(A_i mid Y = y) = sum_i mathbb{E}(X mid Y = y, A_i)mathbb{P}(A_i mid Y = y).$$
So
$$mathbb{E}(X mid Y = y) = mathbb{E}(X mid Y = y, Y = Y_1) mathbb{P}(Y = Y_1 mid Y = y) + mathbb{E}(X mid Y = y, Y = Y_2) mathbb{P}(Y = Y_2 mid Y = y).$$
We can use Bayes' Theorem to calculate the probabilities above:
$$mathbb{P}(Y = Y_1 mid Y = y) = frac{f_{Y_1}(y) mathbb{P}(Y = Y_1)}{f_{Y_1}(y) mathbb{P}(Y = Y_1) + f_{Y_2}(y) mathbb{P}(Y = Y_2)}$$
where
$$f_{Y_i}(y) = frac{1}{sqrt{2pi sigma_i^2}}e^{-y^2/2sigma_i^2} text{for } i = 1, 2.$$
So
$$mathbb{P}(Y = Y_1 mid Y = y) = frac{1/sigma_1 e^{-y^2/2sigma_1^2} mu}{1/sigma_1 e^{-y^2/2sigma_1^2} mu + 1/sigma_2 e^{-y^2/2sigma_2^2} (1 -mu)} = frac{sigma_2 mu e^{-y^2/2sigma_1^2}}{sigma_2 mu e^{-y^2/2sigma_1^2} + sigma_1 (1 - mu)e^{-y^2/2sigma_2^2}}.$$
Also
$$mathbb{P}(Y = Y_2 mid Y = y) = frac{sigma_1 (1 - mu) e^{-y^2/2sigma_2^2}}{sigma_2 mu e^{-y^2/2sigma_1^2} + sigma_1 (1 - mu)e^{-y^2/2sigma_2^2}}.$$
The conditional distribution of $X mid Y = y, Y = Y_i$ is given by
$$X mid Y = y, Y = Y_i sim Nleft(mu_x + rho_ifrac{sigma_x}{sigma_i}(y - mu_i), sigma_x^2 (1 - rho_i^2) right)$$
where $rho_i$ is the correlation of $X$ and $Y_i$, $mu_x = mathbb{E}(X) = 0$ and $mu_i = mathbb{E}(Y_i) = 0$.
Putting this together we have
$$mathbb{E}(X mid Y = y) = rho_1frac{sigma_x}{sigma_1}y cdot p_1 + rho_2frac{sigma_x}{sigma_2}y cdot p_2 = mu_{x mid y},$$
where $p_i = mathbb{P}(Y = Y_i mid Y = y)$.
Returning to the conditional variance formula, we now expand it, conditioning on $Y = Y_i$ again:
$$mathbb{V}(X mid Y = y) = mathbb{E}((X - mu_{xmid y})^{2}mid Y = y)$$
$$= mathbb{E}((X - mu_{xmid y})^{2}mid Y = y, Y = Y_1)mathbb{P}(Y = Y_1 mid Y = y) + mathbb{E}((X - mu_{xmid y})^{2}mid Y = y, Y = Y_2)mathbb{P}(Y = Y_2 mid Y = y).$$
Now $$(X - mu_{xmid y})^{2} = (X - mu_{x mid y,i} + mu_{x mid y,i} - mu_{xmid y})^{2} = (X - mu_{x mid y,i})^2 + 2(X - mu_{x mid y,i})(mu_{x mid y,i} - mu_{xmid y}) + (mu_{x mid y,i} - mu_{xmid y})^2,$$
where $mu_{x mid y,i} = mathbb{E}(X mid Y = y, Y = Y_i)$, so
$$mathbb{E}((X - mu_{xmid y})^{2}mid Y = y = Y_i) = mathbb{E}((X - mu_{x mid y,i})^2 + 2(X - mu_{x mid y,i})(mu_{x mid y,i} - mu_{xmid y}) + (mu_{x mid y,i} - mu_{xmid y})^2mid Y = y = Y_i)$$
$$= sigma_x^2 (1 - rho_i^2) + 0 + (mu_{x mid y,i} - mu_{xmid y})^2.$$
Therefore
$$mathbb{V}(X mid Y = y) = left(sigma_x^2 (1 - rho_1^2) + (mu_{x mid y,1} - mu_{xmid y})^2 right)p_1 + left(sigma_x^2 (1 - rho_2^2) + (mu_{x mid y,2} - mu_{xmid y})^2 right)p_2.$$
$$ = sigma_x^2 + left(- sigma_x^2 rho_1^2 + (mu_{x mid y,1} - mu_{xmid y})^2 right)p_1 + left(- sigma_x^2 rho_2^2 + (mu_{x mid y,2} - mu_{xmid y})^2 right)p_2.$$
$endgroup$
1
$begingroup$
Thanks for your effort, Alex, really appreciate that.
$endgroup$
– user229519
Jan 23 at 20:31
add a comment |
$begingroup$
You can use the Conditional Variance Formula:
$$mathbb{V}(X mid Y = y) = mathbb{E}((X - mathbb{E}(Xmid Y = y))^{2}mid Y = y)$$
We need to calculate $mathbb{E}(X mid Y = y)$, and we can do this using a variation of the Law of Total Expectation. Let $A_i$ be the event $Y = Y_i$.
$$mathbb{E}(X mid Y = y) = int xthinspace f_{x mid y}dx = int xfrac{f_{x,y}}{f_y}dx = int xsum_i frac{f_{x,y,A_i}}{f_y}dx$$
$$= int xsum_i frac{f_{x,y,A_i}}{f_{y,A_i}}frac{f_{y,A_i}}{f_{y}}dx= int xsum_i frac{f_{x,y,A_i}}{f_{y,A_i}}frac{f_{y,A_i}}{f_{y}}dx = sum_iint x thinspace f_{x mid y, A_i}mathbb{P}(A_i mid Y = y)dx$$
$$= sum_i int x thinspace f_{x mid y, A_i}dx cdot mathbb{P}(A_i mid Y = y) = sum_i mathbb{E}(X mid Y = y, A_i)mathbb{P}(A_i mid Y = y).$$
So
$$mathbb{E}(X mid Y = y) = mathbb{E}(X mid Y = y, Y = Y_1) mathbb{P}(Y = Y_1 mid Y = y) + mathbb{E}(X mid Y = y, Y = Y_2) mathbb{P}(Y = Y_2 mid Y = y).$$
We can use Bayes' Theorem to calculate the probabilities above:
$$mathbb{P}(Y = Y_1 mid Y = y) = frac{f_{Y_1}(y) mathbb{P}(Y = Y_1)}{f_{Y_1}(y) mathbb{P}(Y = Y_1) + f_{Y_2}(y) mathbb{P}(Y = Y_2)}$$
where
$$f_{Y_i}(y) = frac{1}{sqrt{2pi sigma_i^2}}e^{-y^2/2sigma_i^2} text{for } i = 1, 2.$$
So
$$mathbb{P}(Y = Y_1 mid Y = y) = frac{1/sigma_1 e^{-y^2/2sigma_1^2} mu}{1/sigma_1 e^{-y^2/2sigma_1^2} mu + 1/sigma_2 e^{-y^2/2sigma_2^2} (1 -mu)} = frac{sigma_2 mu e^{-y^2/2sigma_1^2}}{sigma_2 mu e^{-y^2/2sigma_1^2} + sigma_1 (1 - mu)e^{-y^2/2sigma_2^2}}.$$
Also
$$mathbb{P}(Y = Y_2 mid Y = y) = frac{sigma_1 (1 - mu) e^{-y^2/2sigma_2^2}}{sigma_2 mu e^{-y^2/2sigma_1^2} + sigma_1 (1 - mu)e^{-y^2/2sigma_2^2}}.$$
The conditional distribution of $X mid Y = y, Y = Y_i$ is given by
$$X mid Y = y, Y = Y_i sim Nleft(mu_x + rho_ifrac{sigma_x}{sigma_i}(y - mu_i), sigma_x^2 (1 - rho_i^2) right)$$
where $rho_i$ is the correlation of $X$ and $Y_i$, $mu_x = mathbb{E}(X) = 0$ and $mu_i = mathbb{E}(Y_i) = 0$.
Putting this together we have
$$mathbb{E}(X mid Y = y) = rho_1frac{sigma_x}{sigma_1}y cdot p_1 + rho_2frac{sigma_x}{sigma_2}y cdot p_2 = mu_{x mid y},$$
where $p_i = mathbb{P}(Y = Y_i mid Y = y)$.
Returning to the conditional variance formula, we now expand it, conditioning on $Y = Y_i$ again:
$$mathbb{V}(X mid Y = y) = mathbb{E}((X - mu_{xmid y})^{2}mid Y = y)$$
$$= mathbb{E}((X - mu_{xmid y})^{2}mid Y = y, Y = Y_1)mathbb{P}(Y = Y_1 mid Y = y) + mathbb{E}((X - mu_{xmid y})^{2}mid Y = y, Y = Y_2)mathbb{P}(Y = Y_2 mid Y = y).$$
Now $$(X - mu_{xmid y})^{2} = (X - mu_{x mid y,i} + mu_{x mid y,i} - mu_{xmid y})^{2} = (X - mu_{x mid y,i})^2 + 2(X - mu_{x mid y,i})(mu_{x mid y,i} - mu_{xmid y}) + (mu_{x mid y,i} - mu_{xmid y})^2,$$
where $mu_{x mid y,i} = mathbb{E}(X mid Y = y, Y = Y_i)$, so
$$mathbb{E}((X - mu_{xmid y})^{2}mid Y = y = Y_i) = mathbb{E}((X - mu_{x mid y,i})^2 + 2(X - mu_{x mid y,i})(mu_{x mid y,i} - mu_{xmid y}) + (mu_{x mid y,i} - mu_{xmid y})^2mid Y = y = Y_i)$$
$$= sigma_x^2 (1 - rho_i^2) + 0 + (mu_{x mid y,i} - mu_{xmid y})^2.$$
Therefore
$$mathbb{V}(X mid Y = y) = left(sigma_x^2 (1 - rho_1^2) + (mu_{x mid y,1} - mu_{xmid y})^2 right)p_1 + left(sigma_x^2 (1 - rho_2^2) + (mu_{x mid y,2} - mu_{xmid y})^2 right)p_2.$$
$$ = sigma_x^2 + left(- sigma_x^2 rho_1^2 + (mu_{x mid y,1} - mu_{xmid y})^2 right)p_1 + left(- sigma_x^2 rho_2^2 + (mu_{x mid y,2} - mu_{xmid y})^2 right)p_2.$$
$endgroup$
You can use the Conditional Variance Formula:
$$mathbb{V}(X mid Y = y) = mathbb{E}((X - mathbb{E}(Xmid Y = y))^{2}mid Y = y)$$
We need to calculate $mathbb{E}(X mid Y = y)$, and we can do this using a variation of the Law of Total Expectation. Let $A_i$ be the event $Y = Y_i$.
$$mathbb{E}(X mid Y = y) = int xthinspace f_{x mid y}dx = int xfrac{f_{x,y}}{f_y}dx = int xsum_i frac{f_{x,y,A_i}}{f_y}dx$$
$$= int xsum_i frac{f_{x,y,A_i}}{f_{y,A_i}}frac{f_{y,A_i}}{f_{y}}dx= int xsum_i frac{f_{x,y,A_i}}{f_{y,A_i}}frac{f_{y,A_i}}{f_{y}}dx = sum_iint x thinspace f_{x mid y, A_i}mathbb{P}(A_i mid Y = y)dx$$
$$= sum_i int x thinspace f_{x mid y, A_i}dx cdot mathbb{P}(A_i mid Y = y) = sum_i mathbb{E}(X mid Y = y, A_i)mathbb{P}(A_i mid Y = y).$$
So
$$mathbb{E}(X mid Y = y) = mathbb{E}(X mid Y = y, Y = Y_1) mathbb{P}(Y = Y_1 mid Y = y) + mathbb{E}(X mid Y = y, Y = Y_2) mathbb{P}(Y = Y_2 mid Y = y).$$
We can use Bayes' Theorem to calculate the probabilities above:
$$mathbb{P}(Y = Y_1 mid Y = y) = frac{f_{Y_1}(y) mathbb{P}(Y = Y_1)}{f_{Y_1}(y) mathbb{P}(Y = Y_1) + f_{Y_2}(y) mathbb{P}(Y = Y_2)}$$
where
$$f_{Y_i}(y) = frac{1}{sqrt{2pi sigma_i^2}}e^{-y^2/2sigma_i^2} text{for } i = 1, 2.$$
So
$$mathbb{P}(Y = Y_1 mid Y = y) = frac{1/sigma_1 e^{-y^2/2sigma_1^2} mu}{1/sigma_1 e^{-y^2/2sigma_1^2} mu + 1/sigma_2 e^{-y^2/2sigma_2^2} (1 -mu)} = frac{sigma_2 mu e^{-y^2/2sigma_1^2}}{sigma_2 mu e^{-y^2/2sigma_1^2} + sigma_1 (1 - mu)e^{-y^2/2sigma_2^2}}.$$
Also
$$mathbb{P}(Y = Y_2 mid Y = y) = frac{sigma_1 (1 - mu) e^{-y^2/2sigma_2^2}}{sigma_2 mu e^{-y^2/2sigma_1^2} + sigma_1 (1 - mu)e^{-y^2/2sigma_2^2}}.$$
The conditional distribution of $X mid Y = y, Y = Y_i$ is given by
$$X mid Y = y, Y = Y_i sim Nleft(mu_x + rho_ifrac{sigma_x}{sigma_i}(y - mu_i), sigma_x^2 (1 - rho_i^2) right)$$
where $rho_i$ is the correlation of $X$ and $Y_i$, $mu_x = mathbb{E}(X) = 0$ and $mu_i = mathbb{E}(Y_i) = 0$.
Putting this together we have
$$mathbb{E}(X mid Y = y) = rho_1frac{sigma_x}{sigma_1}y cdot p_1 + rho_2frac{sigma_x}{sigma_2}y cdot p_2 = mu_{x mid y},$$
where $p_i = mathbb{P}(Y = Y_i mid Y = y)$.
Returning to the conditional variance formula, we now expand it, conditioning on $Y = Y_i$ again:
$$mathbb{V}(X mid Y = y) = mathbb{E}((X - mu_{xmid y})^{2}mid Y = y)$$
$$= mathbb{E}((X - mu_{xmid y})^{2}mid Y = y, Y = Y_1)mathbb{P}(Y = Y_1 mid Y = y) + mathbb{E}((X - mu_{xmid y})^{2}mid Y = y, Y = Y_2)mathbb{P}(Y = Y_2 mid Y = y).$$
Now $$(X - mu_{xmid y})^{2} = (X - mu_{x mid y,i} + mu_{x mid y,i} - mu_{xmid y})^{2} = (X - mu_{x mid y,i})^2 + 2(X - mu_{x mid y,i})(mu_{x mid y,i} - mu_{xmid y}) + (mu_{x mid y,i} - mu_{xmid y})^2,$$
where $mu_{x mid y,i} = mathbb{E}(X mid Y = y, Y = Y_i)$, so
$$mathbb{E}((X - mu_{xmid y})^{2}mid Y = y = Y_i) = mathbb{E}((X - mu_{x mid y,i})^2 + 2(X - mu_{x mid y,i})(mu_{x mid y,i} - mu_{xmid y}) + (mu_{x mid y,i} - mu_{xmid y})^2mid Y = y = Y_i)$$
$$= sigma_x^2 (1 - rho_i^2) + 0 + (mu_{x mid y,i} - mu_{xmid y})^2.$$
Therefore
$$mathbb{V}(X mid Y = y) = left(sigma_x^2 (1 - rho_1^2) + (mu_{x mid y,1} - mu_{xmid y})^2 right)p_1 + left(sigma_x^2 (1 - rho_2^2) + (mu_{x mid y,2} - mu_{xmid y})^2 right)p_2.$$
$$ = sigma_x^2 + left(- sigma_x^2 rho_1^2 + (mu_{x mid y,1} - mu_{xmid y})^2 right)p_1 + left(- sigma_x^2 rho_2^2 + (mu_{x mid y,2} - mu_{xmid y})^2 right)p_2.$$
answered Jan 23 at 7:45


AlexAlex
674412
674412
1
$begingroup$
Thanks for your effort, Alex, really appreciate that.
$endgroup$
– user229519
Jan 23 at 20:31
add a comment |
1
$begingroup$
Thanks for your effort, Alex, really appreciate that.
$endgroup$
– user229519
Jan 23 at 20:31
1
1
$begingroup$
Thanks for your effort, Alex, really appreciate that.
$endgroup$
– user229519
Jan 23 at 20:31
$begingroup$
Thanks for your effort, Alex, really appreciate that.
$endgroup$
– user229519
Jan 23 at 20:31
add a comment |
Thanks for contributing an answer to Mathematics Stack Exchange!
- Please be sure to answer the question. Provide details and share your research!
But avoid …
- Asking for help, clarification, or responding to other answers.
- Making statements based on opinion; back them up with references or personal experience.
Use MathJax to format equations. MathJax reference.
To learn more, see our tips on writing great answers.
Sign up or log in
StackExchange.ready(function () {
StackExchange.helpers.onClickDraftSave('#login-link');
});
Sign up using Google
Sign up using Facebook
Sign up using Email and Password
Post as a guest
Required, but never shown
StackExchange.ready(
function () {
StackExchange.openid.initPostLogin('.new-post-login', 'https%3a%2f%2fmath.stackexchange.com%2fquestions%2f3082490%2fconditional-variance-when-there-is-uncertainity-about-the-distribution-of-condit%23new-answer', 'question_page');
}
);
Post as a guest
Required, but never shown
Sign up or log in
StackExchange.ready(function () {
StackExchange.helpers.onClickDraftSave('#login-link');
});
Sign up using Google
Sign up using Facebook
Sign up using Email and Password
Post as a guest
Required, but never shown
Sign up or log in
StackExchange.ready(function () {
StackExchange.helpers.onClickDraftSave('#login-link');
});
Sign up using Google
Sign up using Facebook
Sign up using Email and Password
Post as a guest
Required, but never shown
Sign up or log in
StackExchange.ready(function () {
StackExchange.helpers.onClickDraftSave('#login-link');
});
Sign up using Google
Sign up using Facebook
Sign up using Email and Password
Sign up using Google
Sign up using Facebook
Sign up using Email and Password
Post as a guest
Required, but never shown
Required, but never shown
Required, but never shown
Required, but never shown
Required, but never shown
Required, but never shown
Required, but never shown
Required, but never shown
Required, but never shown
UpwSveaiL IMn lT6wWakZ7Gt
$begingroup$
I think we need more information to solve this problem: what is the joint distribution of $X$ and $Y_1$ (and $X$ with $Y_2$)? We need to know the distribution of $X$ given $Y = y, Y =Y_1$ (and $Y = y, Y =Y_2$) in order to solve this question. Can we assume that it is a bivariate normal distribution? From the information that you've given so far we can't: this post gives an example where $X, Y$ are both normal and have non-zero covariance but are not bivariate normal stats.stackexchange.com/questions/293818/…
$endgroup$
– Alex
Jan 22 at 8:14
$begingroup$
$X$ and $Y_1$, and $X$ and $Y_2$ are both bivariate normal distribution. But when $y$ is realized it is not known whether it comes from $Y_1$ or $Y_2$. Is this information enough, @Alex.
$endgroup$
– user229519
Jan 22 at 14:14