Find optimal Bayesian decision based on a criterion for the posterior mean
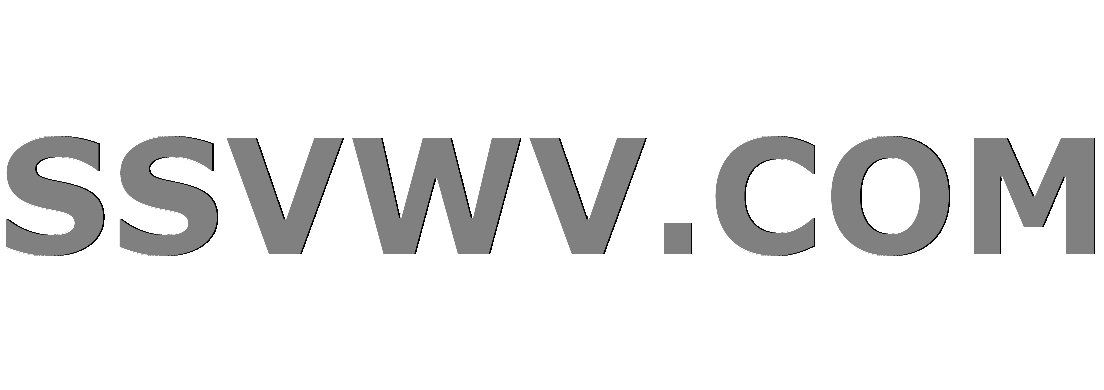
Multi tool use
$begingroup$
I recently ran into the following exercise when practicing for an exam I have about Statistical Inference. The question looks very large and complex and I'm wondering if this is actually true or if it can be solved relatively easily.
The question:
At a critical stage in the development of a new airplane, a decision
must be taken to continue or to abandon the project. The financial
viability of the project can be measured by the parameter
$thetain(0,1)$, the project being profitable if $theta>1/2$. Data
$x$ provide information about $theta$. If $theta<1/2$, the cost of continuing the project is $1/2-theta$, whereas if $theta>1/2$ it is zero. If $theta>1/2$ the cost of abandoning the project is $theta-1/2$, whereas if
$theta<1/2$ it is zero.
We denote the decision to continue the project by $d_1$ and the
decision to abandon the project by $d_2$.
Show that the optimal Bayesian decision is to continue the project if
the posterior mean of $theta$ is greater than $1/2$
My attempt: The general start to problems where we have to find Bayesian decision rules is to compute the loss functions. I found the following loss functions:
$L(theta, d_1) = begin{cases}0 &text{ if }theta > 1/2\1/2 - theta &text{ if }theta < 1/2end{cases},,,L(theta, d_2) = begin{cases}theta - 1/2 &text{ if }theta > 1/2\0 &text{ if } theta < 1/2end{cases}$
Which will allow us to compute the risk functions and this in turn could help us find the decision rule minimizes the Bayes risk, and this will be the Bayes decision rule. I'm not able to get much further than that though, since we have this tricky criterion based on the posterior mean.
How can I solve this exercise? Any tips/help are appreciated.
statistics probability-distributions statistical-inference bayesian descriptive-statistics
$endgroup$
add a comment |
$begingroup$
I recently ran into the following exercise when practicing for an exam I have about Statistical Inference. The question looks very large and complex and I'm wondering if this is actually true or if it can be solved relatively easily.
The question:
At a critical stage in the development of a new airplane, a decision
must be taken to continue or to abandon the project. The financial
viability of the project can be measured by the parameter
$thetain(0,1)$, the project being profitable if $theta>1/2$. Data
$x$ provide information about $theta$. If $theta<1/2$, the cost of continuing the project is $1/2-theta$, whereas if $theta>1/2$ it is zero. If $theta>1/2$ the cost of abandoning the project is $theta-1/2$, whereas if
$theta<1/2$ it is zero.
We denote the decision to continue the project by $d_1$ and the
decision to abandon the project by $d_2$.
Show that the optimal Bayesian decision is to continue the project if
the posterior mean of $theta$ is greater than $1/2$
My attempt: The general start to problems where we have to find Bayesian decision rules is to compute the loss functions. I found the following loss functions:
$L(theta, d_1) = begin{cases}0 &text{ if }theta > 1/2\1/2 - theta &text{ if }theta < 1/2end{cases},,,L(theta, d_2) = begin{cases}theta - 1/2 &text{ if }theta > 1/2\0 &text{ if } theta < 1/2end{cases}$
Which will allow us to compute the risk functions and this in turn could help us find the decision rule minimizes the Bayes risk, and this will be the Bayes decision rule. I'm not able to get much further than that though, since we have this tricky criterion based on the posterior mean.
How can I solve this exercise? Any tips/help are appreciated.
statistics probability-distributions statistical-inference bayesian descriptive-statistics
$endgroup$
1
$begingroup$
I gave a full answer below but if you just want a hint, think about when the expected posterior loss under $d_1$, $E(L(theta, d_1)mid x) = int L(theta, d_1) p(theta mid x) dtheta$, is less than $E(L(theta, d_2)mid x)$.
$endgroup$
– Alex
Jan 8 at 23:10
add a comment |
$begingroup$
I recently ran into the following exercise when practicing for an exam I have about Statistical Inference. The question looks very large and complex and I'm wondering if this is actually true or if it can be solved relatively easily.
The question:
At a critical stage in the development of a new airplane, a decision
must be taken to continue or to abandon the project. The financial
viability of the project can be measured by the parameter
$thetain(0,1)$, the project being profitable if $theta>1/2$. Data
$x$ provide information about $theta$. If $theta<1/2$, the cost of continuing the project is $1/2-theta$, whereas if $theta>1/2$ it is zero. If $theta>1/2$ the cost of abandoning the project is $theta-1/2$, whereas if
$theta<1/2$ it is zero.
We denote the decision to continue the project by $d_1$ and the
decision to abandon the project by $d_2$.
Show that the optimal Bayesian decision is to continue the project if
the posterior mean of $theta$ is greater than $1/2$
My attempt: The general start to problems where we have to find Bayesian decision rules is to compute the loss functions. I found the following loss functions:
$L(theta, d_1) = begin{cases}0 &text{ if }theta > 1/2\1/2 - theta &text{ if }theta < 1/2end{cases},,,L(theta, d_2) = begin{cases}theta - 1/2 &text{ if }theta > 1/2\0 &text{ if } theta < 1/2end{cases}$
Which will allow us to compute the risk functions and this in turn could help us find the decision rule minimizes the Bayes risk, and this will be the Bayes decision rule. I'm not able to get much further than that though, since we have this tricky criterion based on the posterior mean.
How can I solve this exercise? Any tips/help are appreciated.
statistics probability-distributions statistical-inference bayesian descriptive-statistics
$endgroup$
I recently ran into the following exercise when practicing for an exam I have about Statistical Inference. The question looks very large and complex and I'm wondering if this is actually true or if it can be solved relatively easily.
The question:
At a critical stage in the development of a new airplane, a decision
must be taken to continue or to abandon the project. The financial
viability of the project can be measured by the parameter
$thetain(0,1)$, the project being profitable if $theta>1/2$. Data
$x$ provide information about $theta$. If $theta<1/2$, the cost of continuing the project is $1/2-theta$, whereas if $theta>1/2$ it is zero. If $theta>1/2$ the cost of abandoning the project is $theta-1/2$, whereas if
$theta<1/2$ it is zero.
We denote the decision to continue the project by $d_1$ and the
decision to abandon the project by $d_2$.
Show that the optimal Bayesian decision is to continue the project if
the posterior mean of $theta$ is greater than $1/2$
My attempt: The general start to problems where we have to find Bayesian decision rules is to compute the loss functions. I found the following loss functions:
$L(theta, d_1) = begin{cases}0 &text{ if }theta > 1/2\1/2 - theta &text{ if }theta < 1/2end{cases},,,L(theta, d_2) = begin{cases}theta - 1/2 &text{ if }theta > 1/2\0 &text{ if } theta < 1/2end{cases}$
Which will allow us to compute the risk functions and this in turn could help us find the decision rule minimizes the Bayes risk, and this will be the Bayes decision rule. I'm not able to get much further than that though, since we have this tricky criterion based on the posterior mean.
How can I solve this exercise? Any tips/help are appreciated.
statistics probability-distributions statistical-inference bayesian descriptive-statistics
statistics probability-distributions statistical-inference bayesian descriptive-statistics
edited Jan 8 at 21:45
S. Crim
asked Jan 8 at 20:42
S. CrimS. Crim
35612
35612
1
$begingroup$
I gave a full answer below but if you just want a hint, think about when the expected posterior loss under $d_1$, $E(L(theta, d_1)mid x) = int L(theta, d_1) p(theta mid x) dtheta$, is less than $E(L(theta, d_2)mid x)$.
$endgroup$
– Alex
Jan 8 at 23:10
add a comment |
1
$begingroup$
I gave a full answer below but if you just want a hint, think about when the expected posterior loss under $d_1$, $E(L(theta, d_1)mid x) = int L(theta, d_1) p(theta mid x) dtheta$, is less than $E(L(theta, d_2)mid x)$.
$endgroup$
– Alex
Jan 8 at 23:10
1
1
$begingroup$
I gave a full answer below but if you just want a hint, think about when the expected posterior loss under $d_1$, $E(L(theta, d_1)mid x) = int L(theta, d_1) p(theta mid x) dtheta$, is less than $E(L(theta, d_2)mid x)$.
$endgroup$
– Alex
Jan 8 at 23:10
$begingroup$
I gave a full answer below but if you just want a hint, think about when the expected posterior loss under $d_1$, $E(L(theta, d_1)mid x) = int L(theta, d_1) p(theta mid x) dtheta$, is less than $E(L(theta, d_2)mid x)$.
$endgroup$
– Alex
Jan 8 at 23:10
add a comment |
1 Answer
1
active
oldest
votes
$begingroup$
The Bayes risk for continuing the project, $E(L(theta, d_1) mid x)$, is given by
$$E(L(theta, d_1) mid x) = int_0^1L(theta, d_1) p(theta mid x) dx = int_0^{1/2}left(frac{1}{2} - thetaright)p(theta mid x) dtheta$$
$$= frac{1}{2}int_0^{1/2} p(theta mid x) dtheta - int_0^{1/2}theta p(theta mid x) dtheta.$$
And the Bayes risk for cancelling the project, $E(L(theta, d_2) mid x)$, is given by
$$E(L(theta, d_2) mid x) = int_0^1L(theta, d_2) p(theta mid x) dx = int_{1/2}^{1}left(theta - frac{1}{2}right)p(theta mid x) dtheta$$
$$= frac{1}{2}int_{1/2}^{1} theta p(theta mid x) dtheta - frac{1}{2}int_0^{1/2} p(theta mid x) dtheta.$$
The Bayes decision rule is to continue the project when the Bayes risk of continuing is less than the risk of cancelling, i.e. when
$$E(L(theta, d_1) mid x) < E(L(theta, d_2) mid x).$$
This is equivalent to
$$frac{1}{2}int_0^{1/2} p(theta mid x) dtheta - int_0^{1/2}theta p(theta mid x) dtheta < int_{1/2}^{1} theta p(theta mid x) dtheta - frac{1}{2}int_{1/2}^{1} p(theta mid x) dtheta,$$
which is equivalent to
$$frac{1}{2}int_0^{1/2} p(theta mid x) dtheta + frac{1}{2}int_{1/2}^{1} p(theta mid x) dtheta < int_0^{1/2}theta p(theta mid x) dtheta + int_{1/2}^{1}theta p(theta mid x) dtheta,$$
which is equivalent to
$$frac{1}{2}int_0^{1} p(theta mid x) dtheta < int_0^{1}theta p(theta mid x) dtheta.$$
We know that $int_0^{1} p(theta mid x) dtheta = 1$, and that $int_0^{1}theta p(theta mid x) dtheta = E(theta mid x)$, so the Bayes decision rule is that we should continue with the project whenever
$$frac{1}{2} < E(theta mid x).$$
$endgroup$
1
$begingroup$
I came so close with your hint but got stuck in the end anyway, so thanks alot for the full answer! Much appreciated!
$endgroup$
– S. Crim
Jan 9 at 14:04
1
$begingroup$
Glad I could help!
$endgroup$
– Alex
Jan 10 at 16:53
add a comment |
Your Answer
StackExchange.ifUsing("editor", function () {
return StackExchange.using("mathjaxEditing", function () {
StackExchange.MarkdownEditor.creationCallbacks.add(function (editor, postfix) {
StackExchange.mathjaxEditing.prepareWmdForMathJax(editor, postfix, [["$", "$"], ["\\(","\\)"]]);
});
});
}, "mathjax-editing");
StackExchange.ready(function() {
var channelOptions = {
tags: "".split(" "),
id: "69"
};
initTagRenderer("".split(" "), "".split(" "), channelOptions);
StackExchange.using("externalEditor", function() {
// Have to fire editor after snippets, if snippets enabled
if (StackExchange.settings.snippets.snippetsEnabled) {
StackExchange.using("snippets", function() {
createEditor();
});
}
else {
createEditor();
}
});
function createEditor() {
StackExchange.prepareEditor({
heartbeatType: 'answer',
autoActivateHeartbeat: false,
convertImagesToLinks: true,
noModals: true,
showLowRepImageUploadWarning: true,
reputationToPostImages: 10,
bindNavPrevention: true,
postfix: "",
imageUploader: {
brandingHtml: "Powered by u003ca class="icon-imgur-white" href="https://imgur.com/"u003eu003c/au003e",
contentPolicyHtml: "User contributions licensed under u003ca href="https://creativecommons.org/licenses/by-sa/3.0/"u003ecc by-sa 3.0 with attribution requiredu003c/au003e u003ca href="https://stackoverflow.com/legal/content-policy"u003e(content policy)u003c/au003e",
allowUrls: true
},
noCode: true, onDemand: true,
discardSelector: ".discard-answer"
,immediatelyShowMarkdownHelp:true
});
}
});
Sign up or log in
StackExchange.ready(function () {
StackExchange.helpers.onClickDraftSave('#login-link');
});
Sign up using Google
Sign up using Facebook
Sign up using Email and Password
Post as a guest
Required, but never shown
StackExchange.ready(
function () {
StackExchange.openid.initPostLogin('.new-post-login', 'https%3a%2f%2fmath.stackexchange.com%2fquestions%2f3066687%2ffind-optimal-bayesian-decision-based-on-a-criterion-for-the-posterior-mean%23new-answer', 'question_page');
}
);
Post as a guest
Required, but never shown
1 Answer
1
active
oldest
votes
1 Answer
1
active
oldest
votes
active
oldest
votes
active
oldest
votes
$begingroup$
The Bayes risk for continuing the project, $E(L(theta, d_1) mid x)$, is given by
$$E(L(theta, d_1) mid x) = int_0^1L(theta, d_1) p(theta mid x) dx = int_0^{1/2}left(frac{1}{2} - thetaright)p(theta mid x) dtheta$$
$$= frac{1}{2}int_0^{1/2} p(theta mid x) dtheta - int_0^{1/2}theta p(theta mid x) dtheta.$$
And the Bayes risk for cancelling the project, $E(L(theta, d_2) mid x)$, is given by
$$E(L(theta, d_2) mid x) = int_0^1L(theta, d_2) p(theta mid x) dx = int_{1/2}^{1}left(theta - frac{1}{2}right)p(theta mid x) dtheta$$
$$= frac{1}{2}int_{1/2}^{1} theta p(theta mid x) dtheta - frac{1}{2}int_0^{1/2} p(theta mid x) dtheta.$$
The Bayes decision rule is to continue the project when the Bayes risk of continuing is less than the risk of cancelling, i.e. when
$$E(L(theta, d_1) mid x) < E(L(theta, d_2) mid x).$$
This is equivalent to
$$frac{1}{2}int_0^{1/2} p(theta mid x) dtheta - int_0^{1/2}theta p(theta mid x) dtheta < int_{1/2}^{1} theta p(theta mid x) dtheta - frac{1}{2}int_{1/2}^{1} p(theta mid x) dtheta,$$
which is equivalent to
$$frac{1}{2}int_0^{1/2} p(theta mid x) dtheta + frac{1}{2}int_{1/2}^{1} p(theta mid x) dtheta < int_0^{1/2}theta p(theta mid x) dtheta + int_{1/2}^{1}theta p(theta mid x) dtheta,$$
which is equivalent to
$$frac{1}{2}int_0^{1} p(theta mid x) dtheta < int_0^{1}theta p(theta mid x) dtheta.$$
We know that $int_0^{1} p(theta mid x) dtheta = 1$, and that $int_0^{1}theta p(theta mid x) dtheta = E(theta mid x)$, so the Bayes decision rule is that we should continue with the project whenever
$$frac{1}{2} < E(theta mid x).$$
$endgroup$
1
$begingroup$
I came so close with your hint but got stuck in the end anyway, so thanks alot for the full answer! Much appreciated!
$endgroup$
– S. Crim
Jan 9 at 14:04
1
$begingroup$
Glad I could help!
$endgroup$
– Alex
Jan 10 at 16:53
add a comment |
$begingroup$
The Bayes risk for continuing the project, $E(L(theta, d_1) mid x)$, is given by
$$E(L(theta, d_1) mid x) = int_0^1L(theta, d_1) p(theta mid x) dx = int_0^{1/2}left(frac{1}{2} - thetaright)p(theta mid x) dtheta$$
$$= frac{1}{2}int_0^{1/2} p(theta mid x) dtheta - int_0^{1/2}theta p(theta mid x) dtheta.$$
And the Bayes risk for cancelling the project, $E(L(theta, d_2) mid x)$, is given by
$$E(L(theta, d_2) mid x) = int_0^1L(theta, d_2) p(theta mid x) dx = int_{1/2}^{1}left(theta - frac{1}{2}right)p(theta mid x) dtheta$$
$$= frac{1}{2}int_{1/2}^{1} theta p(theta mid x) dtheta - frac{1}{2}int_0^{1/2} p(theta mid x) dtheta.$$
The Bayes decision rule is to continue the project when the Bayes risk of continuing is less than the risk of cancelling, i.e. when
$$E(L(theta, d_1) mid x) < E(L(theta, d_2) mid x).$$
This is equivalent to
$$frac{1}{2}int_0^{1/2} p(theta mid x) dtheta - int_0^{1/2}theta p(theta mid x) dtheta < int_{1/2}^{1} theta p(theta mid x) dtheta - frac{1}{2}int_{1/2}^{1} p(theta mid x) dtheta,$$
which is equivalent to
$$frac{1}{2}int_0^{1/2} p(theta mid x) dtheta + frac{1}{2}int_{1/2}^{1} p(theta mid x) dtheta < int_0^{1/2}theta p(theta mid x) dtheta + int_{1/2}^{1}theta p(theta mid x) dtheta,$$
which is equivalent to
$$frac{1}{2}int_0^{1} p(theta mid x) dtheta < int_0^{1}theta p(theta mid x) dtheta.$$
We know that $int_0^{1} p(theta mid x) dtheta = 1$, and that $int_0^{1}theta p(theta mid x) dtheta = E(theta mid x)$, so the Bayes decision rule is that we should continue with the project whenever
$$frac{1}{2} < E(theta mid x).$$
$endgroup$
1
$begingroup$
I came so close with your hint but got stuck in the end anyway, so thanks alot for the full answer! Much appreciated!
$endgroup$
– S. Crim
Jan 9 at 14:04
1
$begingroup$
Glad I could help!
$endgroup$
– Alex
Jan 10 at 16:53
add a comment |
$begingroup$
The Bayes risk for continuing the project, $E(L(theta, d_1) mid x)$, is given by
$$E(L(theta, d_1) mid x) = int_0^1L(theta, d_1) p(theta mid x) dx = int_0^{1/2}left(frac{1}{2} - thetaright)p(theta mid x) dtheta$$
$$= frac{1}{2}int_0^{1/2} p(theta mid x) dtheta - int_0^{1/2}theta p(theta mid x) dtheta.$$
And the Bayes risk for cancelling the project, $E(L(theta, d_2) mid x)$, is given by
$$E(L(theta, d_2) mid x) = int_0^1L(theta, d_2) p(theta mid x) dx = int_{1/2}^{1}left(theta - frac{1}{2}right)p(theta mid x) dtheta$$
$$= frac{1}{2}int_{1/2}^{1} theta p(theta mid x) dtheta - frac{1}{2}int_0^{1/2} p(theta mid x) dtheta.$$
The Bayes decision rule is to continue the project when the Bayes risk of continuing is less than the risk of cancelling, i.e. when
$$E(L(theta, d_1) mid x) < E(L(theta, d_2) mid x).$$
This is equivalent to
$$frac{1}{2}int_0^{1/2} p(theta mid x) dtheta - int_0^{1/2}theta p(theta mid x) dtheta < int_{1/2}^{1} theta p(theta mid x) dtheta - frac{1}{2}int_{1/2}^{1} p(theta mid x) dtheta,$$
which is equivalent to
$$frac{1}{2}int_0^{1/2} p(theta mid x) dtheta + frac{1}{2}int_{1/2}^{1} p(theta mid x) dtheta < int_0^{1/2}theta p(theta mid x) dtheta + int_{1/2}^{1}theta p(theta mid x) dtheta,$$
which is equivalent to
$$frac{1}{2}int_0^{1} p(theta mid x) dtheta < int_0^{1}theta p(theta mid x) dtheta.$$
We know that $int_0^{1} p(theta mid x) dtheta = 1$, and that $int_0^{1}theta p(theta mid x) dtheta = E(theta mid x)$, so the Bayes decision rule is that we should continue with the project whenever
$$frac{1}{2} < E(theta mid x).$$
$endgroup$
The Bayes risk for continuing the project, $E(L(theta, d_1) mid x)$, is given by
$$E(L(theta, d_1) mid x) = int_0^1L(theta, d_1) p(theta mid x) dx = int_0^{1/2}left(frac{1}{2} - thetaright)p(theta mid x) dtheta$$
$$= frac{1}{2}int_0^{1/2} p(theta mid x) dtheta - int_0^{1/2}theta p(theta mid x) dtheta.$$
And the Bayes risk for cancelling the project, $E(L(theta, d_2) mid x)$, is given by
$$E(L(theta, d_2) mid x) = int_0^1L(theta, d_2) p(theta mid x) dx = int_{1/2}^{1}left(theta - frac{1}{2}right)p(theta mid x) dtheta$$
$$= frac{1}{2}int_{1/2}^{1} theta p(theta mid x) dtheta - frac{1}{2}int_0^{1/2} p(theta mid x) dtheta.$$
The Bayes decision rule is to continue the project when the Bayes risk of continuing is less than the risk of cancelling, i.e. when
$$E(L(theta, d_1) mid x) < E(L(theta, d_2) mid x).$$
This is equivalent to
$$frac{1}{2}int_0^{1/2} p(theta mid x) dtheta - int_0^{1/2}theta p(theta mid x) dtheta < int_{1/2}^{1} theta p(theta mid x) dtheta - frac{1}{2}int_{1/2}^{1} p(theta mid x) dtheta,$$
which is equivalent to
$$frac{1}{2}int_0^{1/2} p(theta mid x) dtheta + frac{1}{2}int_{1/2}^{1} p(theta mid x) dtheta < int_0^{1/2}theta p(theta mid x) dtheta + int_{1/2}^{1}theta p(theta mid x) dtheta,$$
which is equivalent to
$$frac{1}{2}int_0^{1} p(theta mid x) dtheta < int_0^{1}theta p(theta mid x) dtheta.$$
We know that $int_0^{1} p(theta mid x) dtheta = 1$, and that $int_0^{1}theta p(theta mid x) dtheta = E(theta mid x)$, so the Bayes decision rule is that we should continue with the project whenever
$$frac{1}{2} < E(theta mid x).$$
edited Jan 8 at 22:31
answered Jan 8 at 22:25


AlexAlex
559312
559312
1
$begingroup$
I came so close with your hint but got stuck in the end anyway, so thanks alot for the full answer! Much appreciated!
$endgroup$
– S. Crim
Jan 9 at 14:04
1
$begingroup$
Glad I could help!
$endgroup$
– Alex
Jan 10 at 16:53
add a comment |
1
$begingroup$
I came so close with your hint but got stuck in the end anyway, so thanks alot for the full answer! Much appreciated!
$endgroup$
– S. Crim
Jan 9 at 14:04
1
$begingroup$
Glad I could help!
$endgroup$
– Alex
Jan 10 at 16:53
1
1
$begingroup$
I came so close with your hint but got stuck in the end anyway, so thanks alot for the full answer! Much appreciated!
$endgroup$
– S. Crim
Jan 9 at 14:04
$begingroup$
I came so close with your hint but got stuck in the end anyway, so thanks alot for the full answer! Much appreciated!
$endgroup$
– S. Crim
Jan 9 at 14:04
1
1
$begingroup$
Glad I could help!
$endgroup$
– Alex
Jan 10 at 16:53
$begingroup$
Glad I could help!
$endgroup$
– Alex
Jan 10 at 16:53
add a comment |
Thanks for contributing an answer to Mathematics Stack Exchange!
- Please be sure to answer the question. Provide details and share your research!
But avoid …
- Asking for help, clarification, or responding to other answers.
- Making statements based on opinion; back them up with references or personal experience.
Use MathJax to format equations. MathJax reference.
To learn more, see our tips on writing great answers.
Sign up or log in
StackExchange.ready(function () {
StackExchange.helpers.onClickDraftSave('#login-link');
});
Sign up using Google
Sign up using Facebook
Sign up using Email and Password
Post as a guest
Required, but never shown
StackExchange.ready(
function () {
StackExchange.openid.initPostLogin('.new-post-login', 'https%3a%2f%2fmath.stackexchange.com%2fquestions%2f3066687%2ffind-optimal-bayesian-decision-based-on-a-criterion-for-the-posterior-mean%23new-answer', 'question_page');
}
);
Post as a guest
Required, but never shown
Sign up or log in
StackExchange.ready(function () {
StackExchange.helpers.onClickDraftSave('#login-link');
});
Sign up using Google
Sign up using Facebook
Sign up using Email and Password
Post as a guest
Required, but never shown
Sign up or log in
StackExchange.ready(function () {
StackExchange.helpers.onClickDraftSave('#login-link');
});
Sign up using Google
Sign up using Facebook
Sign up using Email and Password
Post as a guest
Required, but never shown
Sign up or log in
StackExchange.ready(function () {
StackExchange.helpers.onClickDraftSave('#login-link');
});
Sign up using Google
Sign up using Facebook
Sign up using Email and Password
Sign up using Google
Sign up using Facebook
Sign up using Email and Password
Post as a guest
Required, but never shown
Required, but never shown
Required, but never shown
Required, but never shown
Required, but never shown
Required, but never shown
Required, but never shown
Required, but never shown
Required, but never shown
9fay6jVp 04wJ9cVunP5cGiQp libZ M4svHpnYR9Gb2nemOpJ4FEU
1
$begingroup$
I gave a full answer below but if you just want a hint, think about when the expected posterior loss under $d_1$, $E(L(theta, d_1)mid x) = int L(theta, d_1) p(theta mid x) dtheta$, is less than $E(L(theta, d_2)mid x)$.
$endgroup$
– Alex
Jan 8 at 23:10